As machine learning obsessives, you might expect us to always pursue the perfect model: the one that takes a new set of system inputs and predicts their outputs correctly every time. In fact, although model accuracy matters, in real-world R&D it is possible to focus on it too much.
A perfect model (even a ‘near-perfect’ one that, say, predicts outputs reliably to within 5%) might mean that machine learning (ML) has cut through multi-dimensional complexity to find a set of robust relationships you had not previously spotted. It’s nice when this is true. But it could also mean that your experiments were trivial or poorly designed and that ML is simply confirming the obvious. In many complex systems with inherent uncertainties such perfection is, in any case, mathematically unachievable.
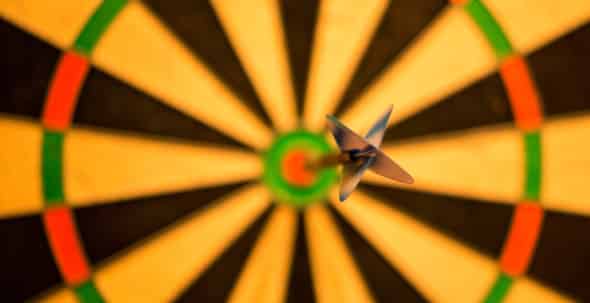
Much more frequently, you want ML to shift the odds in your favour with predictions that outperform the logic currently driving your work. You are usually working with systems where visualising the range of possibilities is beyond the capacity of the human brain and where even relatively sophisticated Design of Experiments methods result in expensive and time-consuming experimental programs. Shifting these odds rarely requires the model to be ‘perfect’. And pursuing the ideal model may waste time that could be better spent elsewhere or, worse, may lead to you inadvertently narrow-down your search space in ways that exclude more creative solutions.
The right question may not be ‘how accurate is my model?’ but one that helps you focus on how useful the model can be. Examples are:
1. ‘Can we get to an answer in fewer experiments?’ Does the ML you are using have the ability to understand what missing data could best improve its accuracy? This information can be used to decide what experiment to do next and the result can be significantly reduced time-to-market. The Alchemite™ software has reduced experimental workloads by 80%+ in some cases. 50% reductions are common.
2. ‘How do we generate new ideas for formulations that achieve our goals?’ Even a moderately-accurate model can generate more new concepts with a chance of success. Where the model comes with a robust estimate of its uncertainty, this can help R&D teams to focus on the predictions that are most likely to work. It can make a big difference if one in every three candidate formulations succeeds when the previous metric was one in five.
3. ‘Can we remove costly or environmentally harmful ingredients?’ Such questions usually come from market, customer, or regulatory pressure and demand a fast response. ML can screen potential solutions and, again, quantifying the uncertainty of the predictions gives an indication of probable success.
4. ‘Where should we focus – which inputs are the most significant?’ The absolute accuracy of predictions may be less important than whether they identify useful relationships, for example between structure, processing variables, and properties. Often, this is the vital information you actually need. Alchemite™ provides a series of analytics tools that enable users to explore the sensitivity of outputs to particular inputs.
5. ‘Can we make better use of the expertise we’ve already developed?’ Very often, insight developed at great expense in R&D projects is never re-used. The ability to capture this insight in an ML model can provide a valuable starting point for future projects.
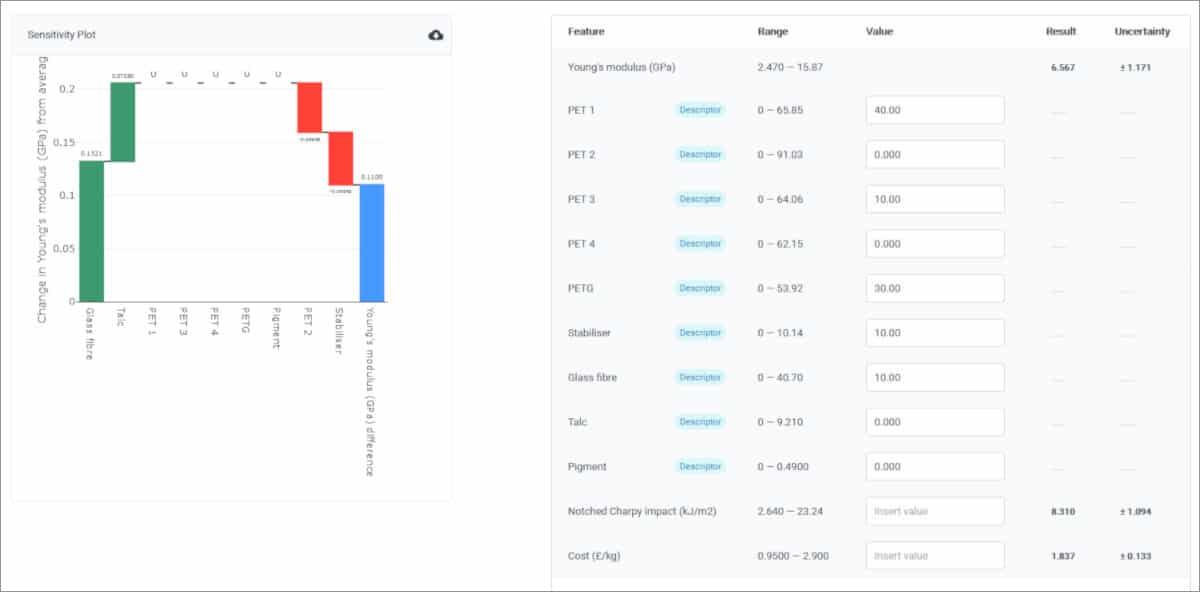
The focus is not on ML as a ‘magic bullet’, but on its use alongside and informing scientific intuition. It’s vital to have the right tools to interrogate and understand the results – such as graphical analytics and uncertainty quantification. This perspective also highlights the importance of being able to quickly generate an ML model, no matter how messy your data. By exploring this model, you gain insight and can improve it iteratively and at relatively low cost.
We still love accurate models. Sometimes, they really do matter. But we also celebrate the spirit of the aphorism often attributed to statistician George Box – “all models are wrong, some are useful.”