Key outcomes
- Discover new relationships between ingredients, process inputs, and release profiles
- Identify the limitations of previous studies
- Perform virtual experiments to test new ideas
- Share data, models, and insights to promote collaboration
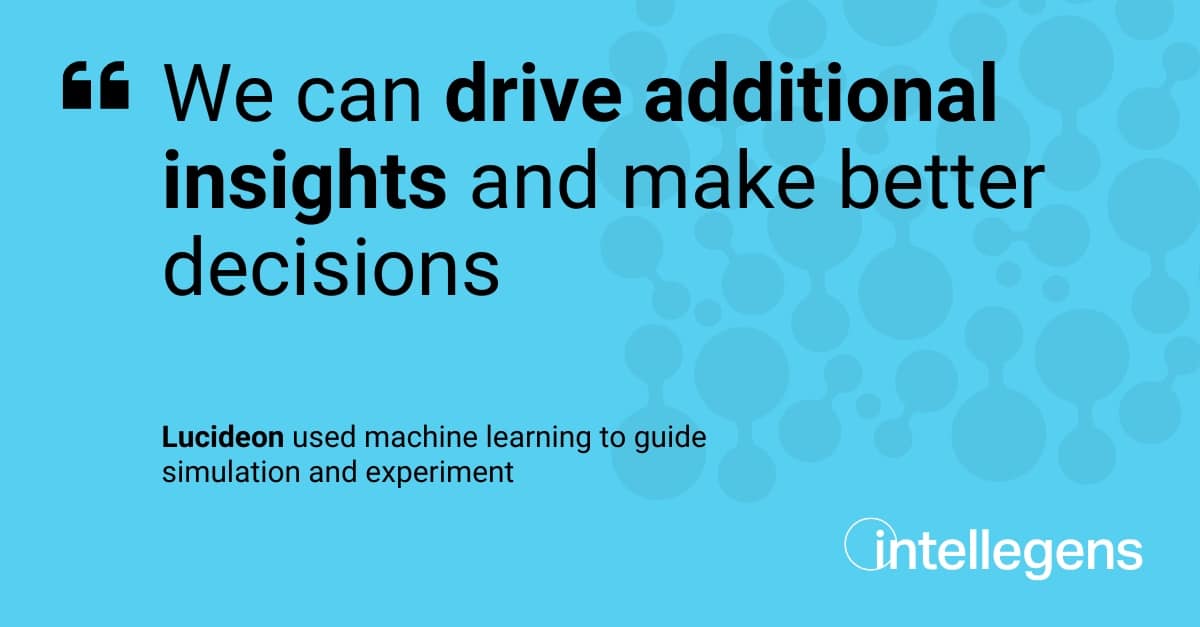
Summary
At an Intellegens webinar, Dr Richard Padbury from Lucideon discussed how machine learning forms part of Lucideon’s vision for transforming materials and process development through an integrated approach that combines data-driven insights with simulation and experiment. He commented “What we’re really excited by is how we can structure and interrogate data, drive additional insights, and make better decisions about which simulations and experiments we ultimately perform.”
Lucideon is a materials technology company with expertise and platform technologies in areas including ceramics, polymers, and controlled release technology. Their broad perspective gives them a good sense of the challenges of developing new materials, where a typical time to go from concept to market can be 10-20 years, and success rates for new ideas are relatively low. Developing and scaling up new materials and processes is a risky business. Lucideon is on the look-out for new tools that can speed up and de-risk this process.
Lucideon exploits the interplay between simulation and experiment – predicting the property of a new material and then using experiment to confirm the predictions. Capabilities in both domains continue to develop dramatically. But both simulation and experiment often come with high costs, and these costs can actually increase as new methods come on-line and more data is generated. Lucideon sees machine learning as a tool to structure and interrogate data in order to drive additional insights and make better decisions about which simulations or experiments to perform.
The challenge is how to navigate the myriad of different data scenarios – particularly those where there are high data volumes or, alternatively, little data or sparse data. High dimensionality, with lots of variables to consider, is also a problem. Overcoming these challenges in the context of materials R&D is what Alchemite™ is designed for. One example of its application was to an inorganic controlled release system, a sparse data scenario, where Lucideon was able to discover relationships in the data, plan the next experiments, and perform virtual experiments to test out new ideas.
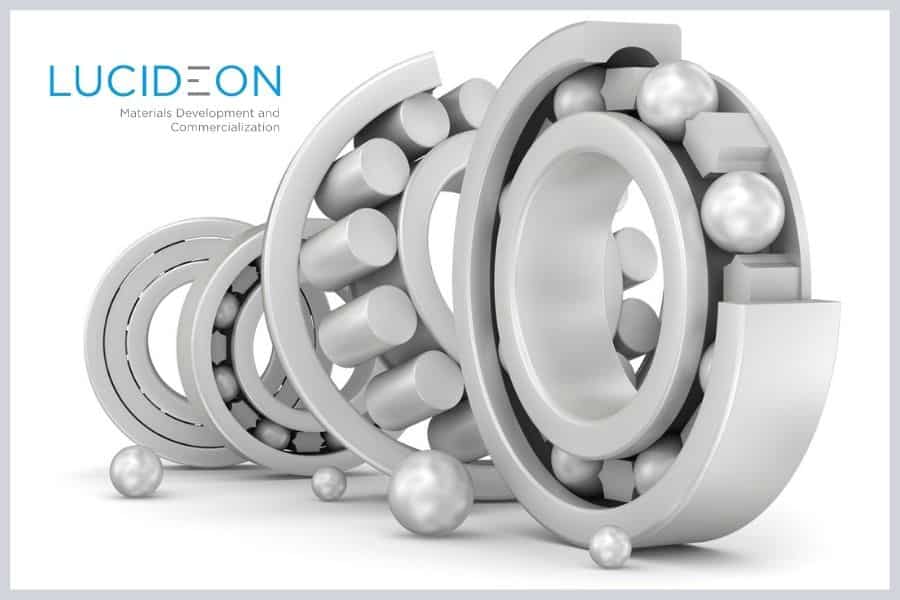