Published paper: Machine learning for batteries
Dec 2021. Published in Cell Reports Physical Science – Alchemite™ machine learning applied to graphite electrodes for lithium-ion batteries.
Key outcomes
- High-coat-weight, graphite-based electrodes were developed for high-energy battery applications
- Both electrode formulation and manufacturing process parameters were optimised
- Alchemite™ machine learning was employed as a predictive tool for cell optimisation
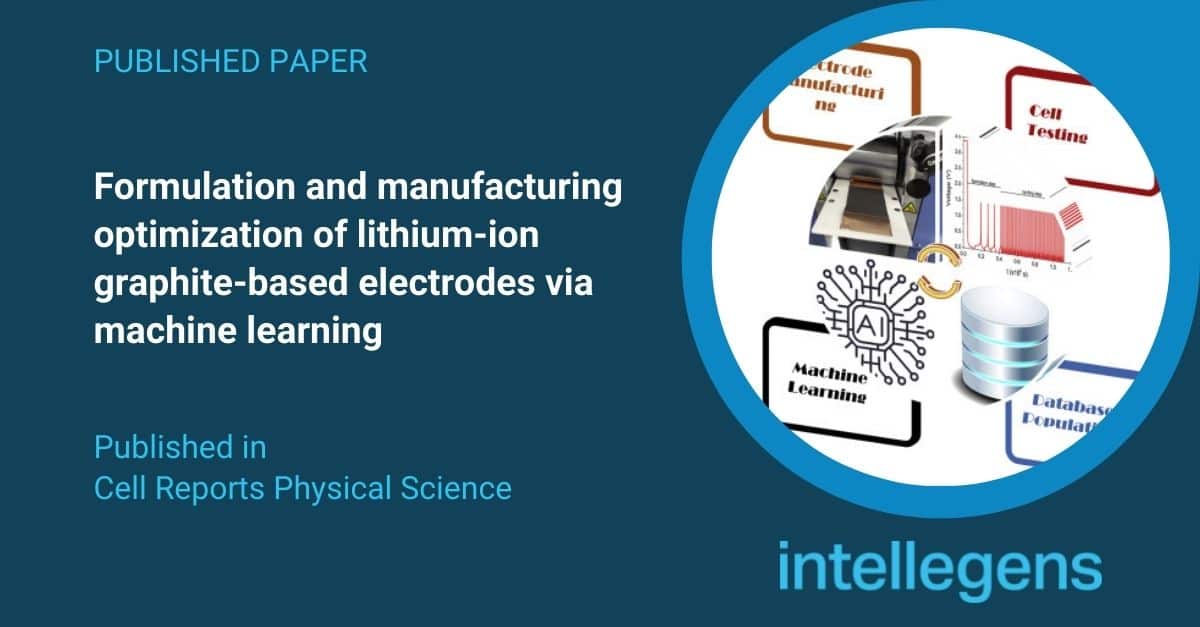
Summary
A collaboration involving Intellegens, the Energy Materials Group at the University of Birmingham, Ansys, and the Faraday Institution applied Alchemite™ machine learning to understand and optimise formulation and manufacturing parameters for lithium-ion battery applications. Such optimisation is critical to enabling higher energy density and longevity in this key class of battery systems, which are essential to applications including electric vehicles.
Based on a dataset of formulations, manufacturing protocols, and performance properties captured in a purpose-built materials database system, a machine learning model was built, validated, and applied to predict the optimal electrode formulation and manufacturing processes. The electrode was manufactured and showed excellent lifecycle and capacity, as predicted.
This work was completed as part of the IDMBAT project, with supporting funding from the Faraday Battery Challenge.
Publication details
Published in: Cell Reports Physical Science 2, 100683 (2021)
Title: Formulation and manufacturing optimisation of lithium-ion graphite-based electrodes via machine learning
DOI: https://doi.org/10.1016/j.xcrp.2021.100683
Authors: Stavros X. Drakopoulos (1), Azarmidokht Gholamipour-Shirazi (1), Paul MacDonald (2), Robert C. Parini (3), Carl D. Reynolds (1) David L. Burnett (1, 4), Ben Pye (1), Kieran B. O’Regan (1,4), Guanmei Wang (2), Thomas M. Whitehead (3), Gareth J. Conduit (3,5), Alexandru Cazacu (2), Emma Kendrick (1)
1. The Energy Materials Group, School of Metallurgy and Materials, University of Birmingham
2. Ansys UK
3. Intellegens
4. The Faraday Institution
5. Theory of Condensed Matter Group, Department of Physics, University of Cambridge[
Downloads
This paper is open access and can be read via the DOI link above.