Additive Manufacturing – always ‘the next big thing’ – is increasingly finding practical applications (as my dentist recently reminded me). But barriers remain to widespread adoption, particularly for applications where part quality or structural performance is critical. Machine learning is helping.
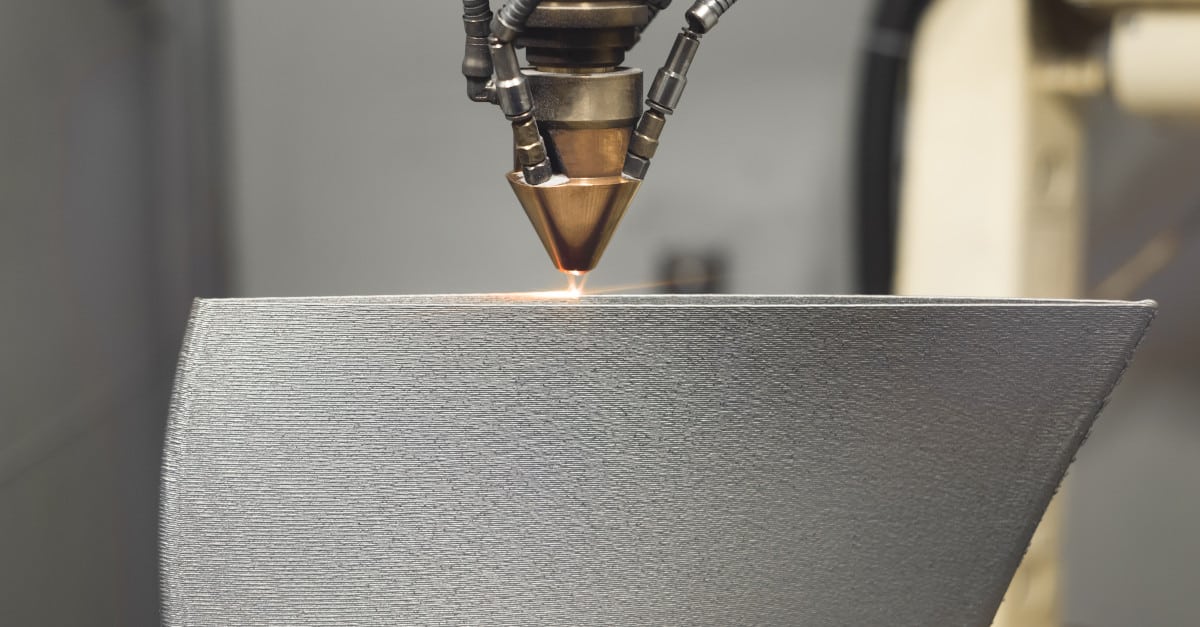
It feels like Additive Manufacturing (AM) has been the next big thing for a while now. A recent chat with my dentist into which he casually dropped a reference to 3D printing of surgical guides reminded me that versions of AM are already widely used. I should have known this, of course, as Lukas Jiranek of Boeing told us in 2021 when discussing an Intellegens machine learning case study in this area: “There are currently over 70,000 AM components flying on Boeing platforms.”
But it’s fair to say that AM is still in pursuit of its full potential, as a recent article from McKinsey reports. It describes AM as ‘on the cusp of stardom’, noting (along with my dentist) its progress in medical applications. But the article highlights four key barriers to the direct, large-scale manufacture of final products across industrial sectors: hardware, software, materials, and services. The challenges of quality and repeatability are particularly acute for parts with structural applications.
At Intellegens, we’ve been involved in the AM materials challenge almost from our inception. The first applications of our Alchemite™ machine learning method included analysing legacy data to propose new alloys for use in AM processes at Rolls-Royce and GKN Aerospace. The results were novel alloy compositions, identified with a substantially reduced need for experimental testing when compared to conventional development approaches. On-going work is exemplified by this recent paper from researchers at Rolls-Royce and the Universities of Cambridge and Sheffield which proposed an alloy for printing onto existing components so that they can be repaired.
What about the hardware, software, and services challenges? These are often around finding the right process parameters to ensure consistent, quality parts in an environment where there can be significant variability between machines and limited data or support to help build understanding. Project teams wanting to be more data-led and to steer away from trial-and-error may want to learn from the test data they already have, enriched with data from prior projects or the literature. But datasets collated in this way are inevitably sparse – not every value will be populated for every possible input or output. This makes them hard to analyse using generic machine learning methods.
The team at Intellegens has now been involved in several projects that address this area, benefiting from the ability of the Alchemite™ machine learning method to learn from sparse datasets. They have been able to understand how factors such as material composition, part geometry, orientation relative to the AM machine, atomisation and laser parameters, and machine output signals impact properties such as porosity, defect area, toughness, elongation, hardness, and cost.
McKinsey in their article proposed a blueprint for AM excellence, and at Intellegens we can see how what they describe as ‘AM centres of competence’ within organisations are beginning to use machine learning in the phases of AM development that McKinsey identifies as: ‘Experiment and partner’ and ‘Build and transfer’, even moving into scale-up.
In March, we’re hosting a webinar with guest speaker Guss Gabe from Lawrence Livermore National Laboratory discussing the use of machine learning for AM process optimisation. And we’ll be at the Additive Manufacturing Users Group (AMUG) event in Chicago. Connect with us at either event and join the discussion about machine learning and the mainstreaming of AM.
Find out more:
Case study – Project MEDAL with AMRC, Boeing, Constellium, GE Additive
Case study – alloy design for heat exchangers at GKN
Case study – alloy design at Rolls-Royce
McKinsey article (March 2022) – The mainstreaming of additive manufacturing