Validating Alchemite™ for AM process optimisation
“I’ve been using Alchemite™ for over a year and my experience has been very good. The web layout is very easy to use and can be picked up by anyone who uses Excel.”
Gabe Guss, Lawrence Livermore National Laboratory
Key outcomes
- Alchemite™ was tested for optimising print parameters for lightweight aerospace parts.
- The machine learning approach compared well to analytical methods previously used for this purpose.
- Alchemite™ was easy to use and will be much easier to apply to new scenarios with many more parameters.
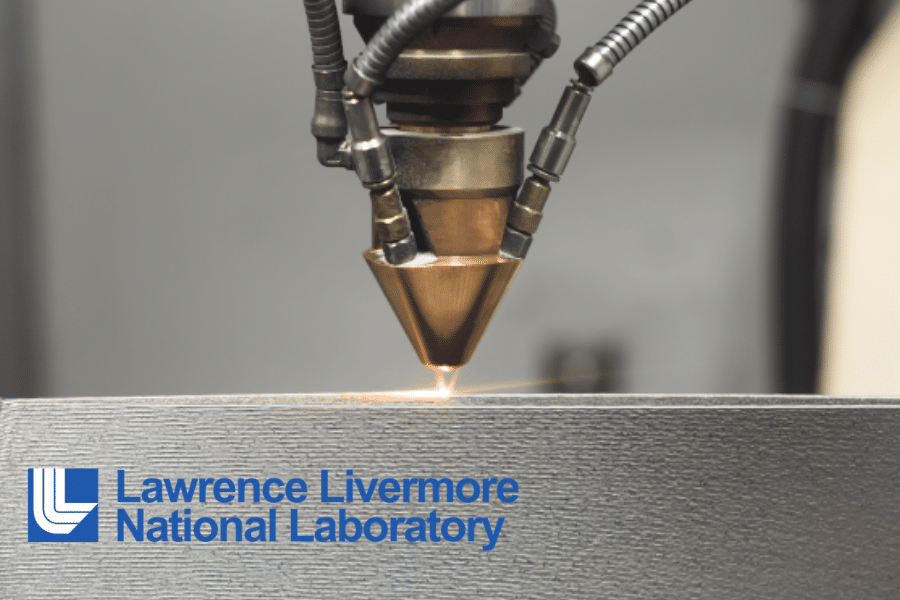
Summary
At a webinar in March 2023, Gabe Guss of Lawrence Livermore National Laboratory presented work to validate the Alchemite™ machine learning software for use in optimising additive manufacturing process parameters. The project focused on Ti-5553 skinned lattices, used in lightweight aerospace parts. The aim was to compare the machine learning method with previous routes to investigate optimal parameters using analytical equations. Could the machine learning be used to build models for predicting porosity and strut size in the part that compared favourably to functional descriptions? Once we have either a model or a function to describe these properties, gradient descent methods can be used to find optimal parameters. The project found that the Alchemite™ machine learning predictions compared well with the analytical methods when both were applied to a relatively simple dataset with just 4 parameters. Although analytical methods were more accurate in some situations, machine learning was easy-to-use and has scope to give rapid answers for new scenarios where the number of parameters increases – in these scenarios, analytical solutions can be time-consuming and difficult to construct. Gabe Guss also emphasised the ease-of-use of the Alchemite™ web browser-based user interface.