“Is AI replacing humans?” It’s an increasingly common, and important, question for society. At Intellegens, we’re often asked whether the aim of our software is to replace scientists in the research process. Not only is our answer an emphatic “No” but we like to point out that our mission is actually the exact opposite: to make machine learning useful by thinking very hard about how it supports those scientists, and about how scientists work with one-another.
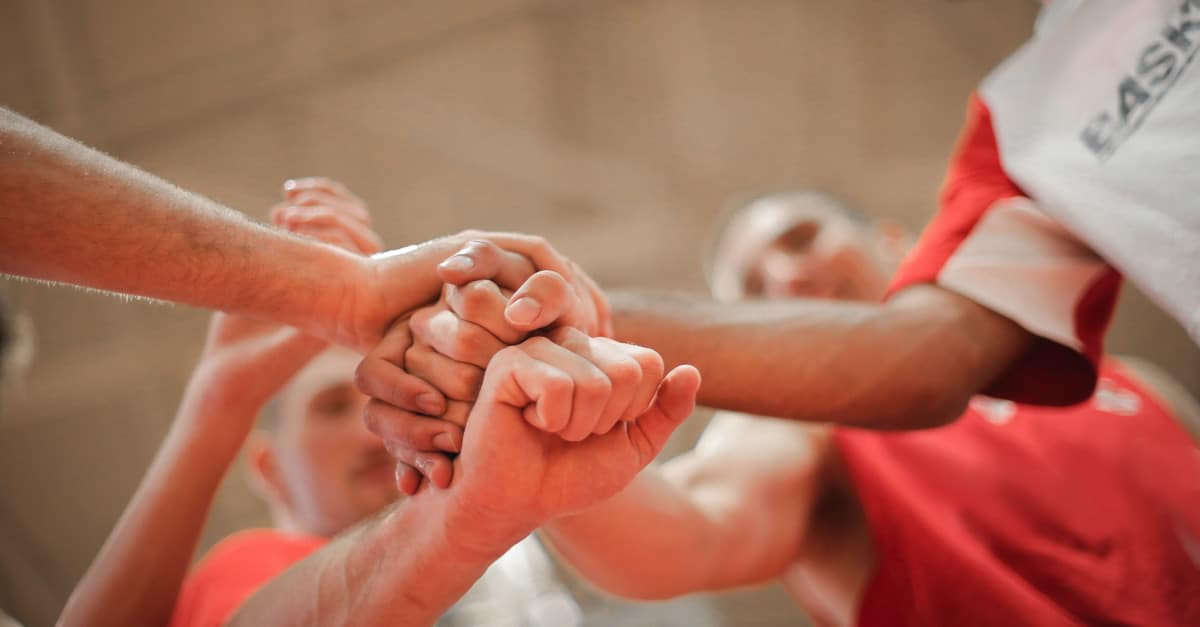
That was very much the theme when our CEO, Ben Pellegrini, opened our User Group Meeting last week. Ben spoke about the need to marry three distinct elements in solving R&D problems: machine learning technology, industry applications, and people. Even the best ML algorithm is of little use in R&D without someone to figure out how to apply it to the most important problems, and of no use at all if it doesn’t then fit with the workflows and priorities of the people tackling those problems. At Intellegens, we reflect this philosophy in the following ways:
1. “It’s the User Experience, stupid.” Great technical products often fail because they’re just too hard to use, and that’s just… well, not smart. At the UGM, we talked about the 5-step design process that we use to refine user experience of our software. It starts and ends with users – working directly with them to understand how they really use Alchemite™ in practice and to refine the software accordingly. Recent changes included the new dashboard view (shown below), which was driven by user demands for a streamlined, high-level perspective of their model and tested with users as it was created.
2. Much more than just software. We want users to be able to just pick up the software and use it (see point 1!). But barriers exist not simply in “knowing which buttons to press”, but in where and how the technology fits into real projects – what to analyse, and how to use the results. That’s why our Alchemite™ Science Team is critical to how we work with customers. They bring experience of projects elsewhere to advise new users (or longstanding users facing a new challenge), helping them to define successful projects and become self-sufficient, quickly. The Team is also available to support projects where in-house resource is constrained.
3. Science as a team game. Intellegens has always been active in industry and scientific collaborations that push the boundaries of applying machine learning and grow collective understanding of how to adopt it. Two recent examples are the ‘Trustworthy AI‘ project, which looks at lowering barriers to applying machine learning in the chemicals and materials sector, and a new collaboration on applying AI to oligonucleotide manufacturing in the life sciences.
The ethical discussions around AI and how it will affect humans, for both good and ill, will continue – and rightly so. But in our corner of the AI universe – machine learning as a productivity tool for R&D – the focus is very much on getting people and the technology to work together, ultimately, to provide scientists with deeper insights and release more of their time to do great science, together.
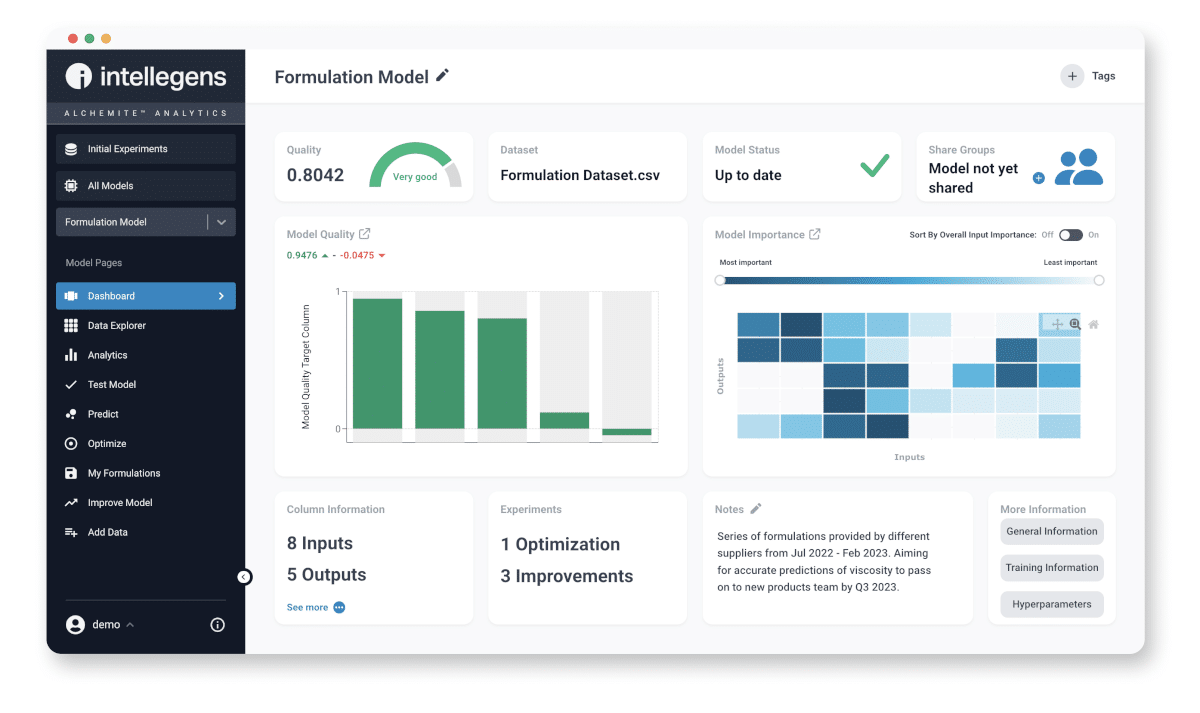