Mario Cordero from Welding Alloys Group gave a compelling presentation at this month’s Intellegens webinar, describing a case study in the development of new materials. And we found one point that he made particularly interesting. Applications that get labelled ‘high tech’ – biotech, aerospace, additive manufacturing – get a lot of attention, much of it justified. But so-called heavy industry, with its reliance on iron alloys and steels, continues to power much of the world economy. And research challenges in this area are equally fascinating and important.
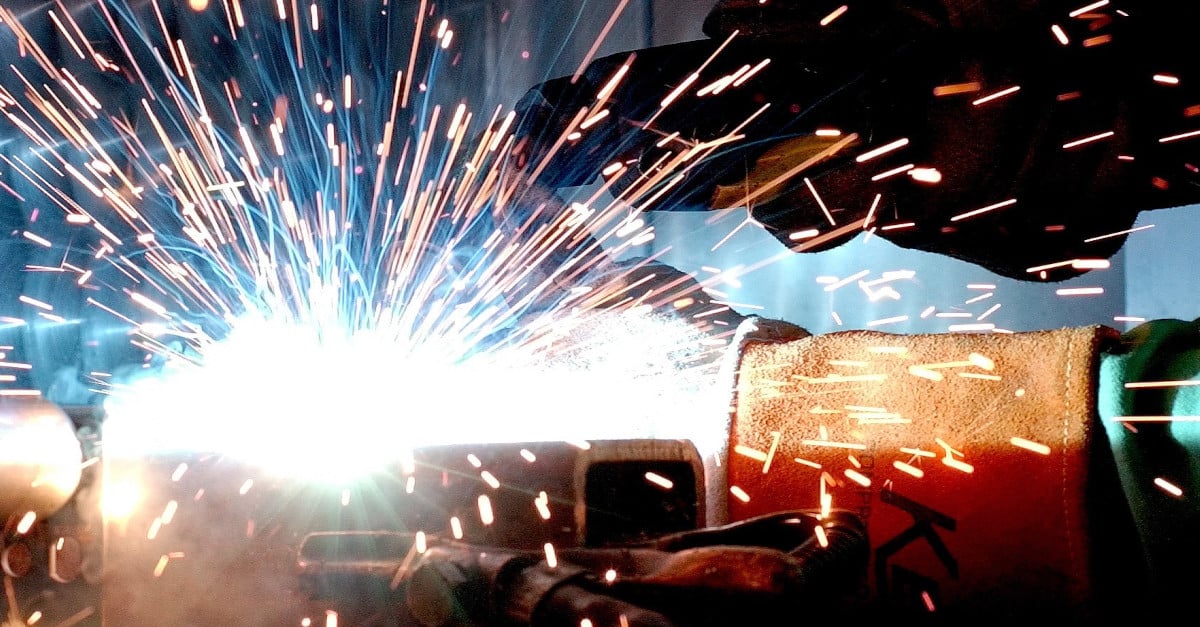
Mario gave two examples during his talk (which you can find recorded, here). One was machinery used to process sugar cane, which needs to be repaired and renewed while it continues to operate. Another was massive heavy digging equipment, with rotors and shafts that required repair and renewal. In both cases, wear was a key factor. In mining alone, one paper estimates that 17% of the consumed energy is used to combat wear failure, accounting for 2.7% of global CO2 emissions. Corrosion, due to chemicals or water, is also a vital factor. According to the World Corrosion Organisation, the estimated annual cost of all forms of corrosion worldwide is $2.2 trillion, 3% of the world’s GDP.
Reducing these costs and extending the lifetime of equipment in heavy industry requires materials innovation – in the materials used, their processing, the surface treatments applied to them, and the way that they are used. And these heavy industries increasingly need to be light on their feet, able to respond to shifting regulations and objectives due to environmental concerns, or to price or supply chain changes in their raw materials. The example that Mario explored was of the need to reduce Chromium content in developing new wear-resistant hardfacing alloys – a response to environmental concerns.
The heritage of these industries does give them one advantage. They typically have large resources of legacy data, collected over decades of material development, testing, and application. The challenge is to extract the maximum insight from this data, particularly when it has been collected over many different projects. Machine learning can play a role, provided that the methods used are able to cope with the sparse datasets (i.e., data with gaps in it) that typically arise when we assemble data from disparate sources. If we can build models from such data, these have a number of valuable applications in heavy industry:
- Gaining insight into materials properties, such as in this study of steels at OCAS.
- Proposing designs for new materials, as in the Welding Alloys Group example.
- Predictive maintenance, in which data about the in-service performance of equipment can be used to plan maintenance schedules, avoiding costly failures.
- Process optimisation, in which machine learning is used to refine process parameters, lowering energy costs and improving quality from all types of process.
When we think of artificial intelligence and machine learning, we automatically think ‘high tech’. It’s useful to realise that some of the industries we may not think of in these terms are actually applying leading-edge technologies, with the potential to make dramatic cost and environmental impacts.