Intellegens publishes peer-reviewed paper with Johnson Matthey
Intellegens and partner Johnson Matthey announced the publication of a peer-reviewed paper, “Accelerating the Design of Automotive Catalysts Products Using Machine Learning”.
Key outcomes
- Alchemite™ accurately modelled 16 key performance targets for catalyst products.
- Routes were proposed to develop more effective products with fewer experiments.
- The proposed formulations are undergoing experimental validation.
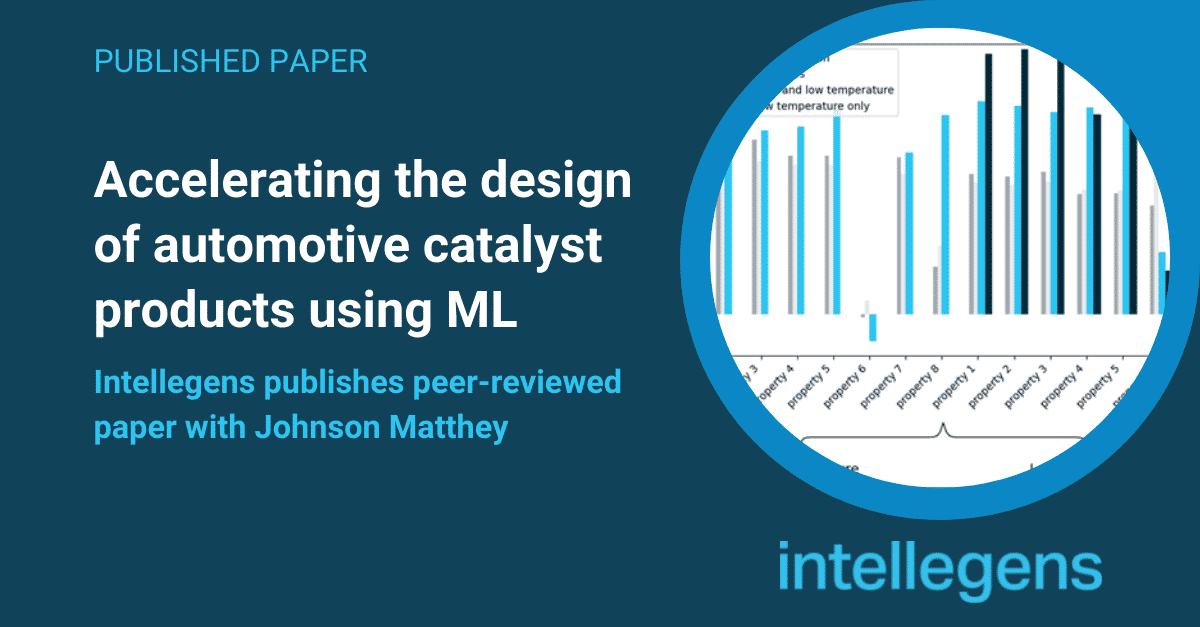
Summary
The design of catalyst products to reduce harmful emissions is currently an intensive process of expert-driven discovery, taking several years to develop a product. Machine learning can accelerate this timescale, leveraging historic experimental data from related products to guide which new formulations and experiments will enable a project to most directly reach its targets. We used machine learning to accurately model 16 key performance targets for catalyst products, enabling detailed understanding of the factors governing catalyst performance and realistic suggestions of future experiments to rapidly develop more effective products. The proposed formulations are currently undergoing experimental validation.
Publication details
Published in: Johnson Matthey Technology Review 66, (2), 130 (2022)
Title: Accelerating the Design of Automotive Catalyst Products Using Machine Learning
Authors: Tom Whitehead (1), Flora Chen (2), Christopher Daly (2), Gareth Conduit (1)
(1) Intellegens
(2) Johnson Matthey