An additive manufacturing case study
- Design AM alloys by understanding key relationships in the data.
- Extract extra value from test data, reducing costs by almost 40% when optimising process parameters.
- Support quality assurance by calculating key performance indicators from large amounts of monitoring data.
- Enable customer service in discussion of downstream processes.
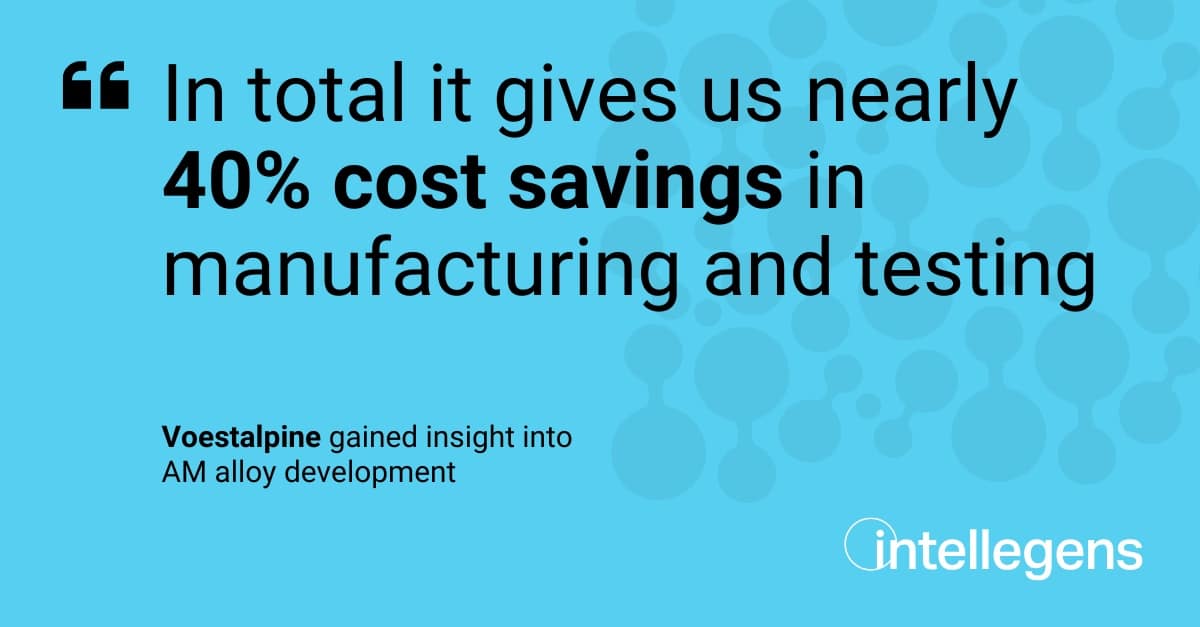
Summary
At an Intellegens webinar, Liang Wu, Process Development Engineer at Voestalpine, outlined their successful use of the Alchemite™ machine learning (ML) software over the previous three years. Voestalpine is a leading steels and technology company with a strong track record in materials and process innovation. In 2021, it began using Alchemite™ in its Additive Manufacturing Center, seeking more insights from data and guidance to focus its R&D, testing, and QA processes.
Liang Wu described the approach to ML at Voestalpine. Domain experts apply Alchemite™ to create ML models focused on specific problems and to refine these models using cycles of design of experiment. The models yield critical insights – for example, which changes in alloy composition are most likely to have a positive impact on target properties. These enable the Voestalpine team to more effectively focus research. Once models are validated, they can also be applied by general technical staff to guide and inform testing, production, and maintenance.
He outlined four specific examples:
Design of new AM alloys. Transfer of materials technologies into Additive Manufacturing often does not work. But ML helps to optimise this process. In one case, it established the importance of a particular martensitic phase in determining key mechanical properties, directing the team to focus on modifying Chromium concentration in a move that led to better alloy performance.
Process parameter development. ML has extracted more value from test data, enabling more efficient optimisation of process parameters. ML models enable one round of testing to provide insights where previously multiple test would have been required. And ML can also capture and study complex relationships between many inputs and outputs. Over three years, Voestalpine have identified almost 40% cost savings due to applying Alchemite™ to testing and manufacturing in this area.
Quality assurance. AM is highly digitalised, with sensors monitoring every aspect and generating overwhelming amounts of data. By feeding this data into ML models that can calculate key performance factors, useful quality assurance reports are created with a lot less effort.
Customer service. ML models can even play a role in enabling more effective business communications with customers. Sharing models can enable customers to explore the possibilities of Voestalpine materials, working with the Voestalpine business team, and to specify their requirements more easily. This also enables Voestalpine R&D scientists to focus on their core research work.
A full recording of the webinar, including Liang Wu’s presentation and a demonstration of the Alchemite™ software for an AM application, is available.
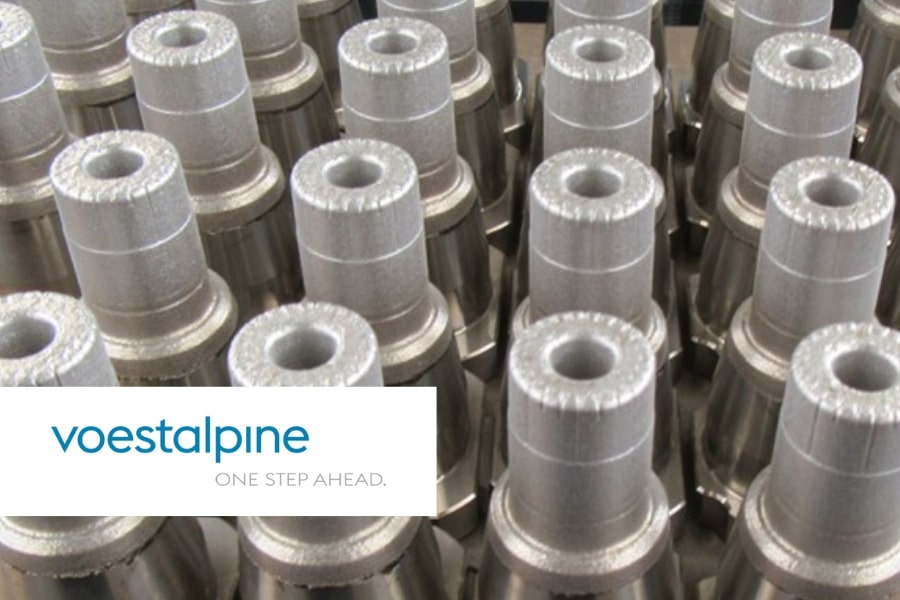