Alchemite™ machine learning has been widely applied in the chemical and process industries. Applications have included development of speciality and fine chemicals, plastics, catalysts, paints and coatings, battery chemistry, lubricants, adhesives, and more.
With Alchemite™, customers in these sectors can learn from their R&D and process data, generating powerful machine learning models that can then be applied to:
- Efficiently design experiments, focusing on the most productive routes to reduce workloads by 50-80%
- Investigate new chemistries and formulations, finding improved solutions while cutting time to market
- Extract maximum value from data, identifying hidden relationships and generating breakthrough insights.
Case study: Johnson Matthey
Johnson Matthey have used Alchemite™ to design catalysts and related processes with applications in clean air and life sciences. Project successes have included a 4% increase in yield on one key process, with significant cost and energy benefits on scale-up, and 50-80% efficiency increases in experimental programmes.
Alchemite™ for formulations
Alchemite™ puts you on the fast track to optimising formulated products – from foods to aerospace coatings, paints to pharmaceuticals, plastics to perfumes. Formulation scientists can rapidly test out new ideas and gain breakthrough insights from their data. Experimentalists can find and explore new combinations while dramatically reducing the number of experiments needed. Apply the power of machine learning through desktop software that is quick to learn and easy to use.
Alchemite™ for Design of Experiments
Alchemite™ is a data-driven experimental design tool that helps your organisation become more productive with no extra effort. Powerful machine learning enables your team to work smarter, reducing experimental time by up to 80%. No lengthy training courses, no need for advanced statistics, and no coding required.
Find out more
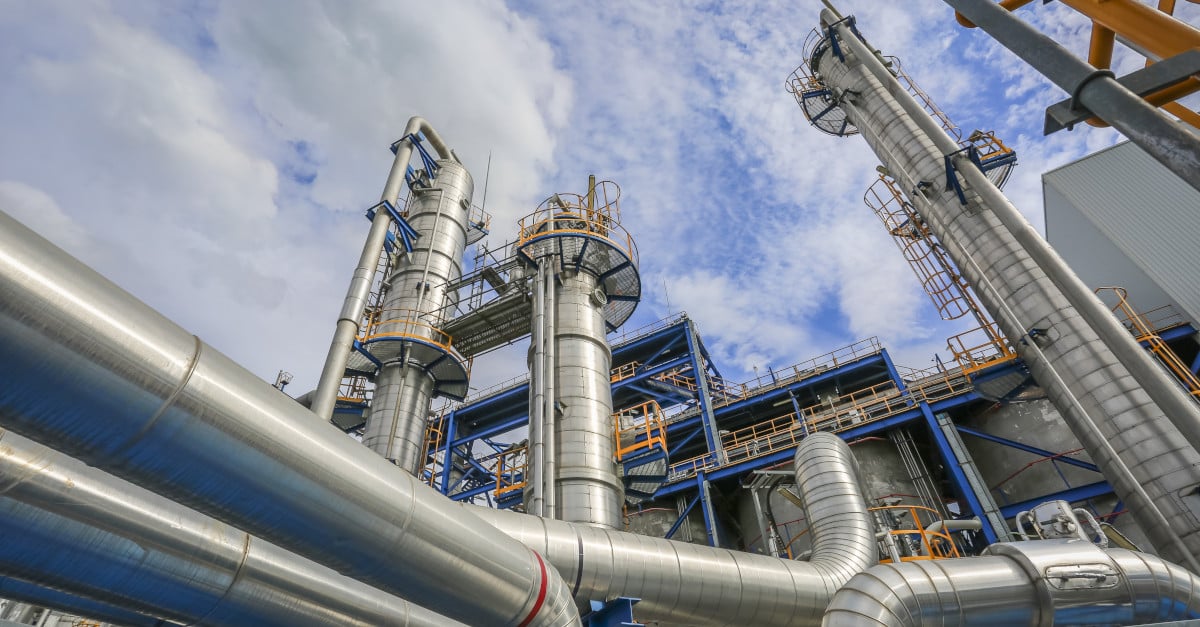