The Alchemite™ technology originated in an alloy development project with Rolls-Royce, and its advanced machine learning has since been applied in areas including additive manufacturing, plastics, composites, and ceramics.
With Alchemite™, customers in these sectors can learn from their R&D and process data, generating powerful machine learning models that can then be applied to:
- Identify new materials design or processing options, cutting time-to-market for innovative solutions
- Efficiently design experiments, focusing on the most productive routes to reduce workloads by 50-80%
- Extract maximum value from data, learning more about critical property – process – structure relationships
Case study: Materials & processes at Voestalpine
Voestalpine is a leading steels and technology company with a strong track record in materials and process innovation. It applies Alchemite™ in its Additive Manufacturing Center, with results including design of new AM alloys, reducing costs by almost 40% when optimising process parameters, support for efficient quality assurance, and enabling customer service in discussion of downstream processes.
Alchemite™ for R&D insights
Unlock the insights hidden in your data through the power of machine learning. In materials discovery and development, Alchemite™ makes it quick and easy to learn from any dataset, creating models that you can apply to fill in missing information, find critical relationships, and guide decision making.
Alchemite™ for materials design
Alchemite™ puts you on the fast track to optimising any product where the ‘formulation problem’ – optimising ingredients and processing conditions to achieve the desired properties – is relevant. This includes not only classic formulated products, such as paints and plastics, but also applications such as alloys, specialist coatings, battery materials, and concrete. Scientists can rapidly test out new ideas and gain breakthrough insights from their data. Experimentalists can find and explore new combinations while dramatically reducing the number of experiments needed. Apply the power of machine learning through desktop software that is quick to learn and easy to use.
Alchemite™ for Design of Experiments
Alchemite™ is a data-driven experimental design tool that helps your organisation become more productive with no extra effort. Powerful machine learning enables your team to work smarter, reducing experimental time by up to 80%. No lengthy training courses, no need for advanced statistics, and no coding required.
Find out more
- Rolls-Royce case study – superalloy design
- AMRC case study – composite manufacturing
- NASA case study – material and component design
- OCAS (Arcelor) case study – steel properties
- Welding Alloys case study – hardfacing consumables
- Lawrence Livermore case study – additive manufacturing
- Blog – Machine learning and the Age of Plastic
- Blog – 10,000+ years of metal innovation
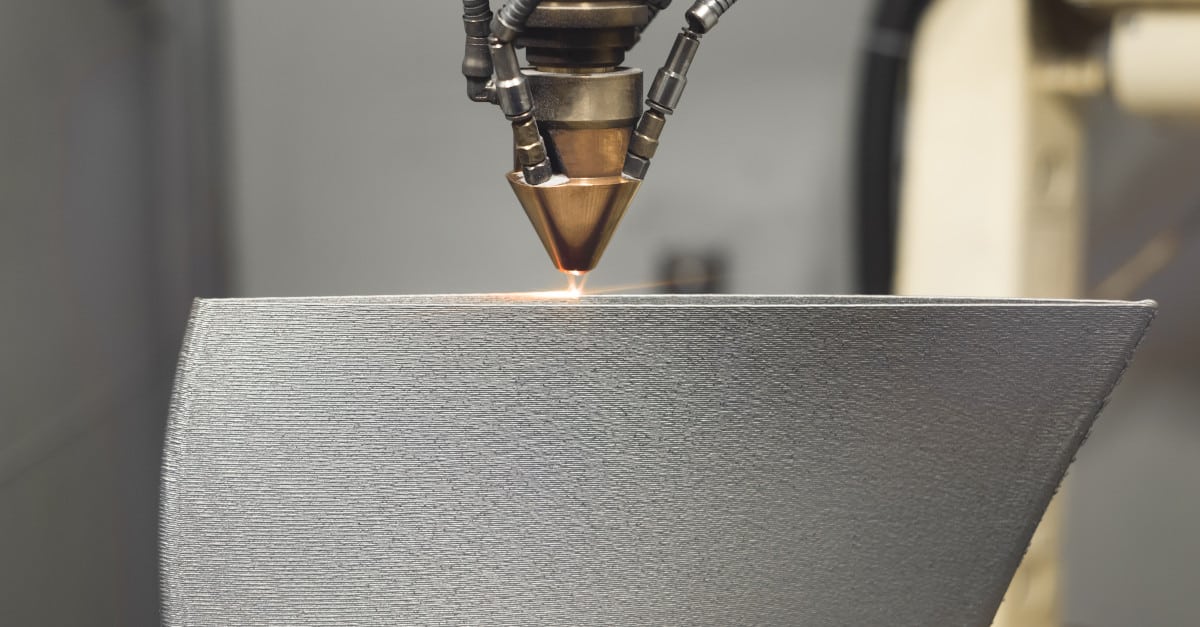