We’ve been digging into Design of Experiments (DOE) on these blogs lately – understanding how machine learning guides experimental programs to optimise formulations, chemistry, or processing parameters. But this approach gives you something in addition – a tool that could be deployed to manage and continuously improve live products and processes.
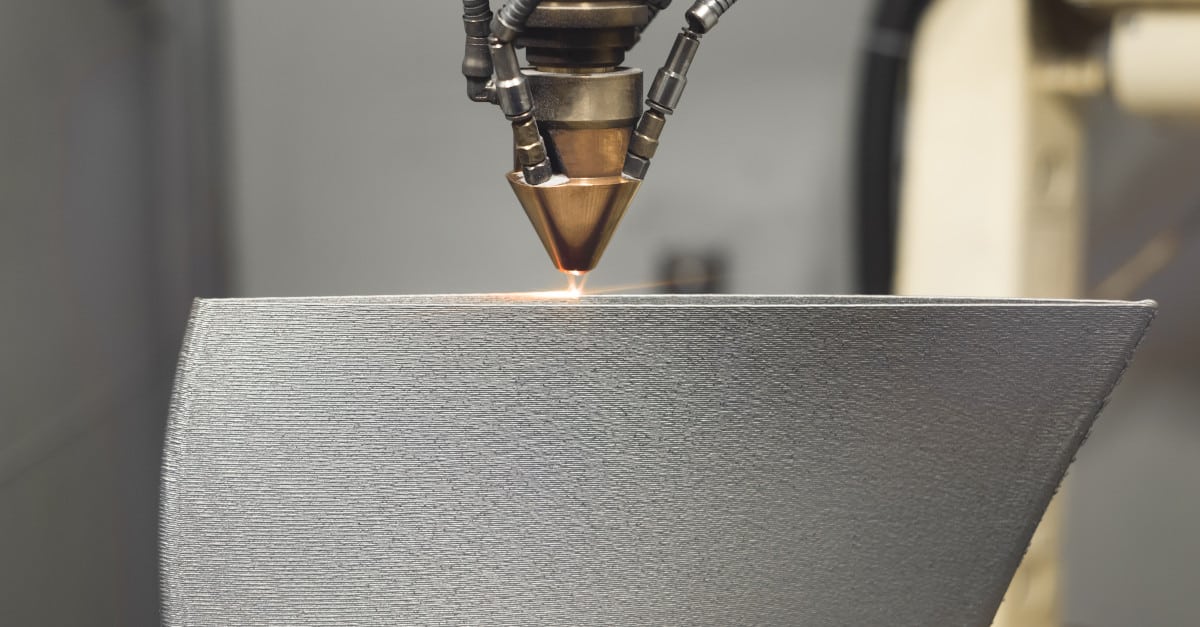
Adaptive DOE using machine learning is based on an iterative approach to experiment that ‘tunes’ a machine learning model, learning from each round of new experimental data to improve its understanding of the system being studied. Success is achieved when the model predicts system behaviour with sufficient accuracy, enabling it to identify the settings that best deliver the target outcomes – for example, the ingredient mix and process settings that give the right combination of shelf-life and texture for a new food product.
But now imagine taking that model and connecting it to the live process so that it can tweak process settings to modify outcomes, while all the time continuing to learn and further improve its modelling. Here’s three examples of such an approach.
Autonomous processes
The first was shared in our recent webinar with Photocentric on supporting autonomous 3D printing. Here, the Alchemite™ software has been used to identify optimal process parameters for different parts being manufactured through an innovative high-speed digital process. Next steps in the project are to connect the models developed through this work to the live machines, so that they can learn and find better process parameters as more parts are made – continuously lowering run-times, costs, and carbon footprint.
On-device
In a second example, Intellegens has been involved in a project to develop a light footprint version of a machine learning model to run on a chip in a consumer device. For this device, a number of inputs – for example, how frequently the device is activated, for how long, and in what orientation – can combine in subtle ways to affect the user experience. The model can take inputs from the operation of the device and use these to select the settings most likely to ensure satisfactory outputs for the user. This scenario is common in, for example, medical device applications.
Providing a service
Finally, a leading provider of a formulated product has taken its use of Alchemite™ machine learning beyond the lab and is providing a machine learning model to its customers via an app. Customers can use the app to fine-tune their use of the product, modifying ingredients and process conditions for results that best match their exact application.
Powered by the API
Such projects are enabled by the Alchemite™ Architect API – the flexible programming interface that makes it easy for other codes and systems to talk to the machine learning model. They are at the cutting-edge of the use of Alchemite™, beyond its now routine use as a day-to-day DOE tool. But they show the potential for machine learning outside the lab – in production, on device, or as a customer service – giving us a glimpse into the future and a reminder that the knowledge captured through adaptive DOE has the potential to provide even greater returns further down the line.
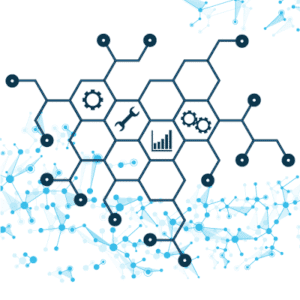