Tooling optimisation for composite drilling using machine learning
- Accurately predict a key failure mechanism based on data from 80% fewer tests than are typically required
- Understand the impact of factors such as tool geometry and material selection
- Inform design of experimental campaigns to remove unnecessary tests
- Cut direct costs and reduce material wastage
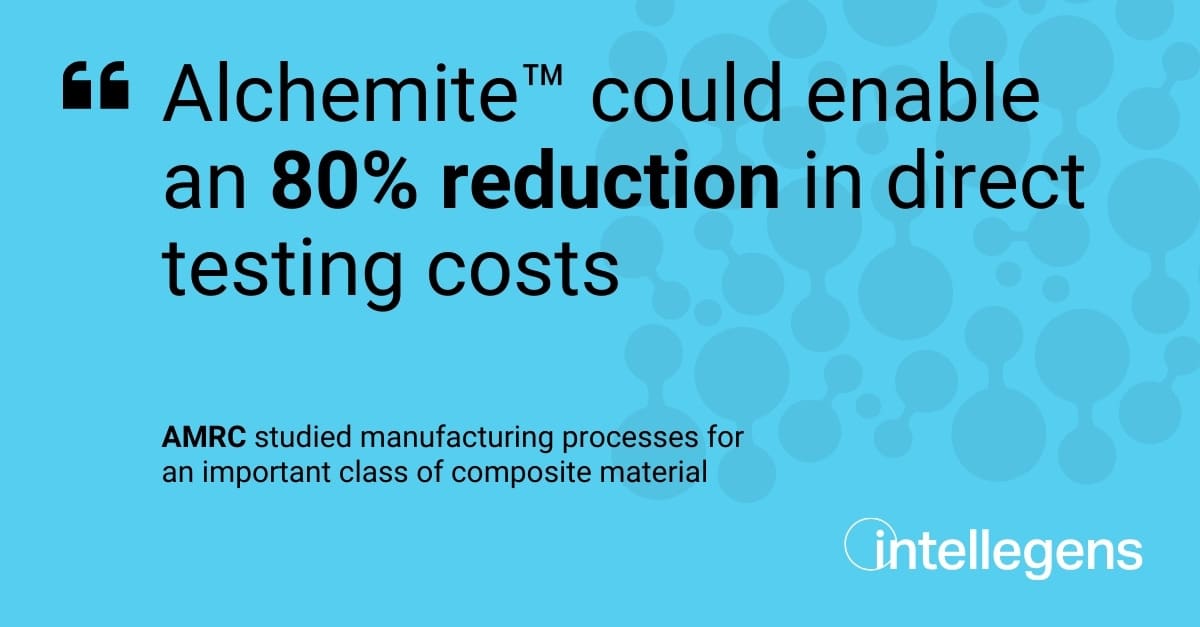
Summary
Alchemite™ was applied at the AMRC to understand manufacturing processes for an important class of composite material used in the aerospace and automotive sectors.
Laminated fibre-reinforced polymer matrix composites are widespread in aerospace and increasingly used in other sectors, such as automotive. Identifying optimal cutting parameters for a given tool-composite pair is of utmost importance, since this can considerably reduce component non-conformance. Surface delamination during machining has been reported to result in 60% of all part rejections during final aircraft assembly. Understanding and controlling delamination requires significant experimental effort, typically involving systematic drilling and analysis of a thousand holes.
The Intellegens machine learning software, Alchemite™, can significantly reduce this experimental time by quantifying complicated nonlinear tool-composite relationships. It enables analysis, understanding, and sharing of complex data relationships, guiding tooling design and selection prior to experimental campaigns. In this study at the University of Sheffield Advanced Manufacturing Centre (AMRC) Alchemite™ delivered useful predictions of future tooling performance from sparse and noisy data based on 80+% fewer experiments and identified irrelevant features for predicting tool performance, facilitating further experimental cost savings.
Related information:
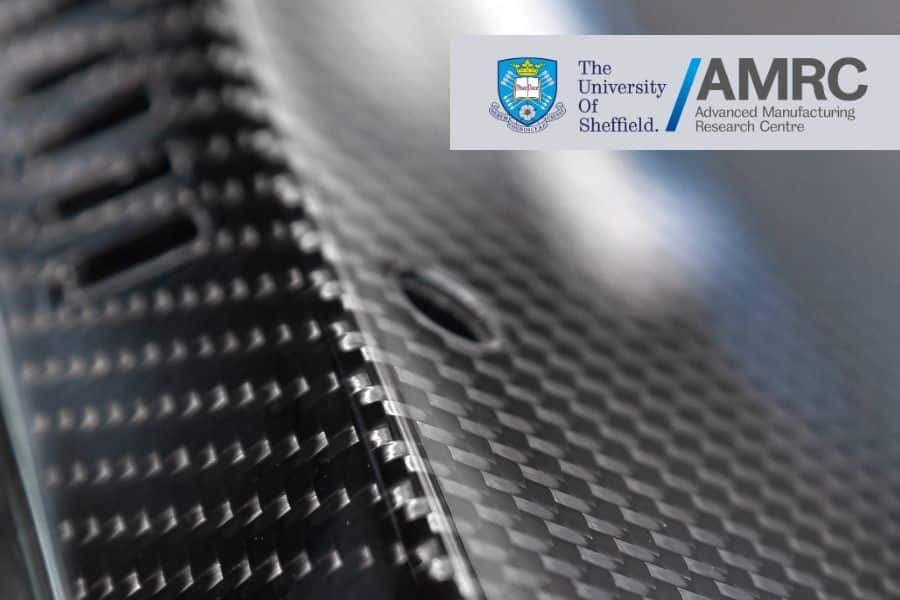