Project MEDAL optimises AM process parameters
This project demonstrated the effectiveness of Alchemite™ machine learning in accelerating process parameter optimisation for a new laser powder bed fusion (LBPF) additive manufacturing process. Such a capability will enable AM process teams to bring new processes to market faster, by significantly reducing the amount of experiment involved.
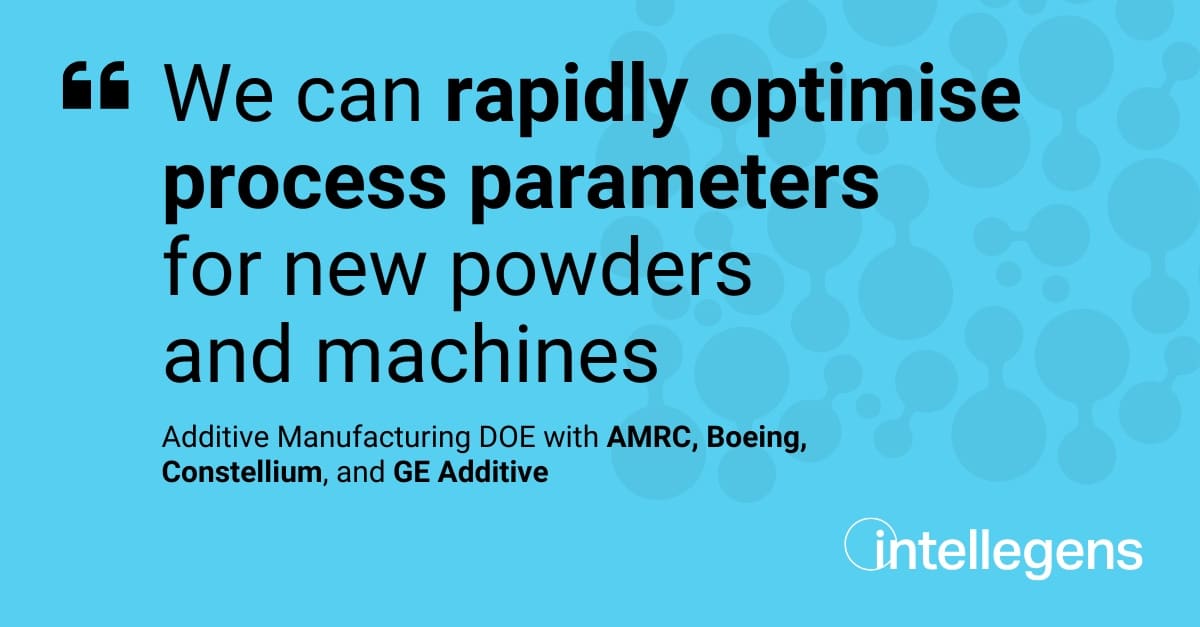
Summary
“Machine learning (ML) has the potential to be a key technology in accelerating further development and adoption of AM”, explains Project MEDAL participant Lukas Jiranek of Boeing. ML can make the additive manufacturing (AM) process for metallic alloys in aerospace cheaper and faster, supporting the production of lightweight, fuel-efficient aircraft.
In collaboration with the University of Sheffield Advanced Manufacturing Research Centre (AMRC) and Boeing, Intellegens applied the Alchemite™ machine learning software to design new AM parameter sets for laser powder bed fusion (LPBF) and test them for nickel base alloys across two experimental cycles. Results from the second cycle showed good agreement with the predictions. The model was then used to develop process parameters for the new additive-specific Aheadd® CP1 powder from Constellium in combination with the 400W M2 machine from GE Additive.
The project team was able to move from the new powder to final parameters in just two builds, while applying no expert statistical knowledge. This shows how Alchemite™ machine learning can dramatically speed up AM process parameter optimisation.
Related information:
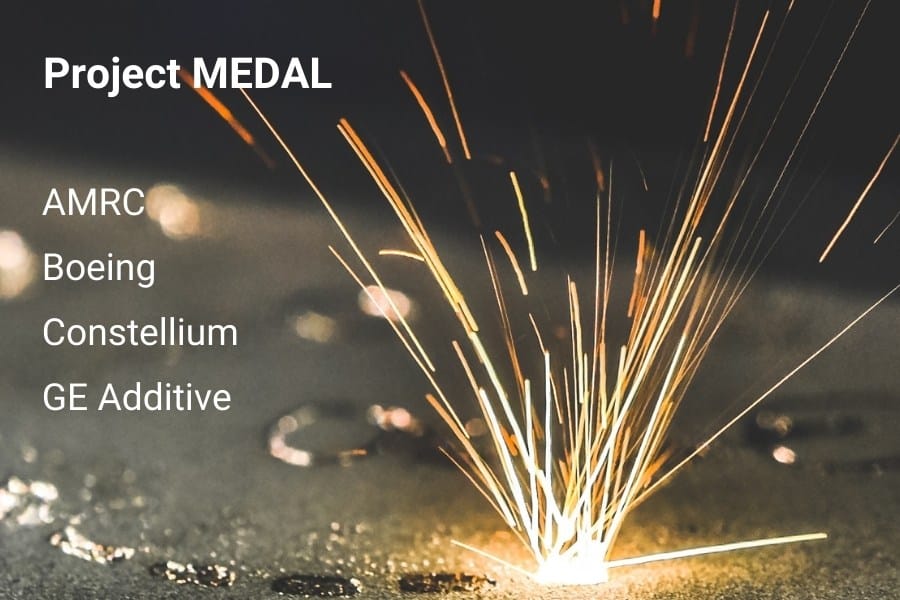