Project in collaboration with Rolls-Royce
The Alchemite™ technology was first validated in a collaboration between Rolls-Royce Aerospace and the University of Cambridge on the computational design of a new nickel-base superalloy for aero engine applications. The project found the material most likely to simultaneously fulfil eleven different physical criteria. This work was published in the journal Materials Design.
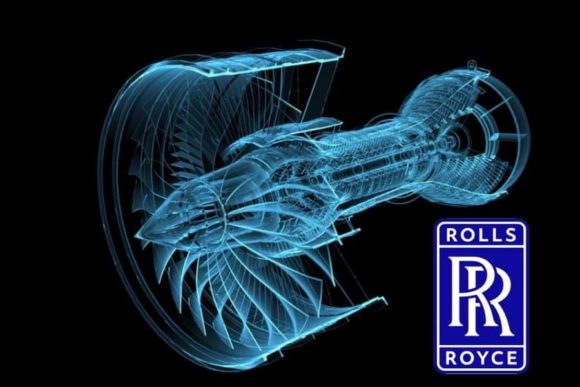
Key outcomes
- Designed a new alloy to satisfy 11 physical criteria that was experimentally-validated to outperform existing alloys.
- Design process involved 90% fewer costly experiments.
- Such a reduction has potential to reduce costs by $millions and cut years from discovery and validation times.
Summary
The Alchemite™ deep learning technology was applied in a research collaboration with Rolls-Royce to model, discover, and optimise new alloys that simultaneously satisfy up to eleven physical criteria for disc applications in aero engines. The artificial neural network was trained from pre-existing materials data, enabling the prediction of individual material properties both as a function of composition and heat treatment routine. By incorporating uncertainty it can find potential alloys with the greatest probability of meeting the design specification. The project designed a new polycrystalline nickel-base superalloy with the optimal combination of cost, density, γ′ phase content and solvus, phase stability, fatigue life, yield stress, ultimate tensile strength, stress rupture, oxidation resistance, and tensile elongation. Experimental data demonstrated that the proposed alloy fulfills the computational predictions, possessing multiple physical properties, particularly oxidation resistance and yield stress, that exceed existing commercially available alloys. The technology has also been used to design a nickel-based alloy for a combustion liner, and two Mo-based alloys for forging tools
Publication details
Publication: Materials & Design 131, 358 (2017)
Title: Design of a nickel-base superalloy using a neural network
Authors: B.D. Conduit, N.G. Jones, H.J. Stone & G.J. Conduit
DOI / Link: https://doi.org/10.1016/j.matdes.2017.06.007