Have you ever…
…done an experiment that wasn’t necessary?
…spent time on a problem that someone had already solved?
…realised that you could have gained a key insight months ago?
Research can be frustrating. Very often, we feel that we should already have the answer we’re looking for. What could we save if we knew what we already know?
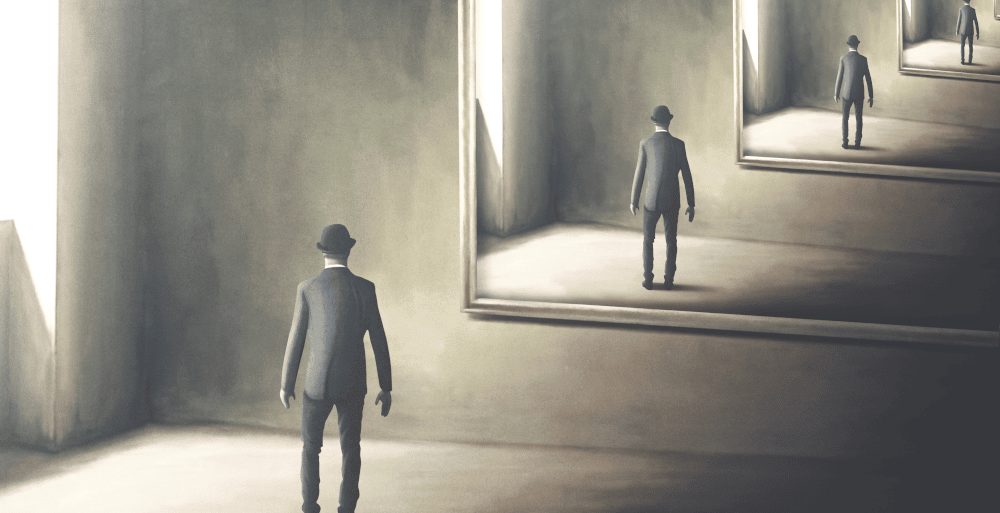
There many reasons R&D organisations don’t maximise the investments that they have already made in knowledge. Here are just three:
Hidden treasure. Important information, for example, key relationships between the inputs and outputs in a formulation project, can lurk unfound in legacy data because the right analysis tools are not applied to it, or because we didn’t know what to look for.
Single-use research. That’s research that we pay for, use once, and then throw away. Projects that generate data for one purpose but have no mechanism to share that data with other projects that could exploit it. Modelling work that generates a great report, but is never evaluated for wider applications.
Lost expertise. As much as 25% of the chemicals industry workforce may be eligible for retirement in the first half of this decade, according to Deloitte. That’s a lot of expertise and knowledge walking out of the door that may not be fully captured for reuse by the next generation of R&D professionals.
What is the machine learning (ML) perspective on this? ML can only be part of an R&D strategy to get more from locked-up information and knowledge. Clearly, R&D organisations need to be looking at their knowledge management and training processes, at the right cheminformatics and materials data management infrastructure, at a full gamut of data analytics capabilities, and much more.
But at Intellegens, we’ve found two ways that ML can be part of the solution.
1. Extracting value from messy experimental or process data. Often, when data is collated from more than one project or source, it is messy. It hasn’t been captured consistently and not all values have been measured in every test. The resulting datasets are sparse (i.e., many values are missing) and noisy. Costly, time-consuming pre-processing is needed to make this data useful. Significant time and effort can be saved if machine learning methods can work directly on sparse, noisy data, to find that ‘hidden treasure’, with much less need for data-cleansing.
2. Capturing knowledge. ML models are able to capture complex, non-linear relationships in systems, so that users can predict and study how changes in the inputs might affect the outputs in otherwise unpredictable ways. Such models are not replacements for years of human experience in formulation design or polymer chemistry. But ML models developed by experts in these areas, appropriately annotated and stored for reuse, can be a valuable tool for passing on knowledge.
We’ve worked with chemical R&D organisations to analyse legacy data. Through our Alchemite™ web platform, we help teams to share and exploit the results of their analysis. Could such approaches be part of your strategy to mine your available data, avoid repeating valuable research, and capture vital expertise? If so, let’s chat!