The only certainty is uncertainty” – American mathematician John Allen Paulos.
Perhaps we all remember a time, maybe at school, when we thought science and mathematics were all about giving us definitive answers. During our careers, as we tackle real-world problems, we understand that this is rarely the case. Almost every system we study, every experiment we conduct, contains uncertainty. This may be natural scatter in the experimental results. It could arise from limitations in the precision of measurement methods, or simply from errors when making these measurements. It might result from having to extrapolate from incomplete data.
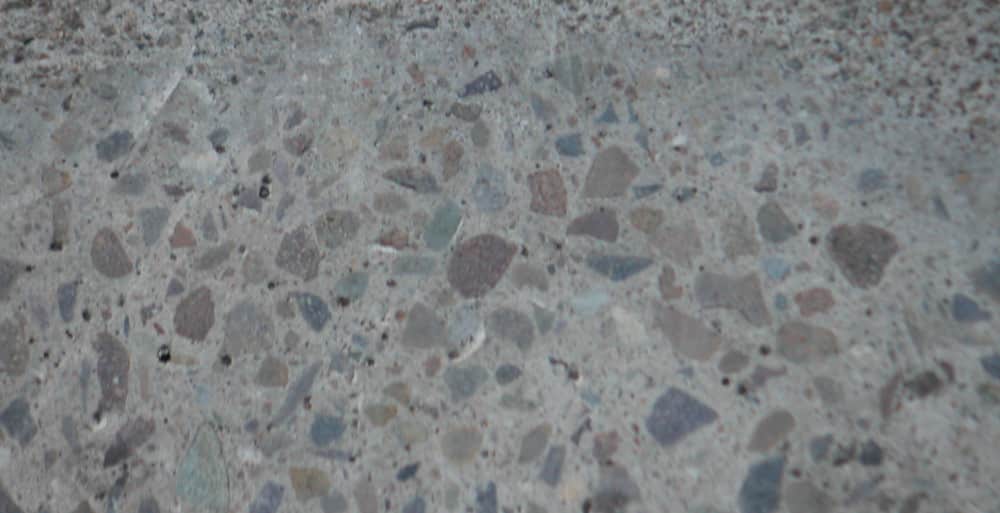
The same applies when we model these systems with machine learning. The predictions will always be uncertain, partly due to inherent scatter in the training data, and partly because we build models from limited data.
We need to embrace this uncertainty. Rather than expecting to eliminate it, we need to understand it, quantify it, and use that information to make better decisions. Indeed, a model containing negligible uncertainty is unlikely to be very useful or insightful, just as a straight line that passes through all points on a graph simply tells us that our system is straightforward to characterise. Uncertainty comes with the hard problems, the ones that are valuable to solve.
This is why, at Intellegens, we’ve invested a lot of effort to ensure that our Alchemite™ algorithm faithfully quantifies the uncertainty in its predictions. It goes beyond conventional methods that, at worst, do not really estimate uncertainty at all but assume a normal distribution, or only capture one of many contributions to the overall uncertainty. Accurate quantification makes it possible to work out, for example, the probability that an experiment will succeed, so the user can target experimental work accordingly.
A recent paper from the research group of Intellegens CSO, Dr Gareth Conduit at the University of Cambridge, goes a step further in embracing uncertainty. The work focused on the design of concrete, in which the random distribution and size of aggregate particles (see image above) means that measurements of many parameters associated with the material appear ‘noisy’. This work did not simply quantify the uncertainty, it got the machine learning method to learn from the noise in the data, finding that the uncertainty in one particular physical parameter could itself be used to help predict the concrete strength. As a result of this work, two new concrete mixes were proposed, made, and found to behave as predicted, exceeding the properties of commercially available mixes.
There is one certainty here: machine learning can do some great things in the world of chemicals and materials. But only if you take the right approach to uncertainty!
Find out more: