By Ben Pellegrini, Intellegens CEO
How can machine learning make Design of Experiments (DOE) easier and deliver better results?
In the first of this short series of blogs, we saw the value of DOE. In the second blog, we saw why conventional DOE often falls short.
Here, we conclude by discussing a new, adaptive approach to DOE, enabled by machine learning.
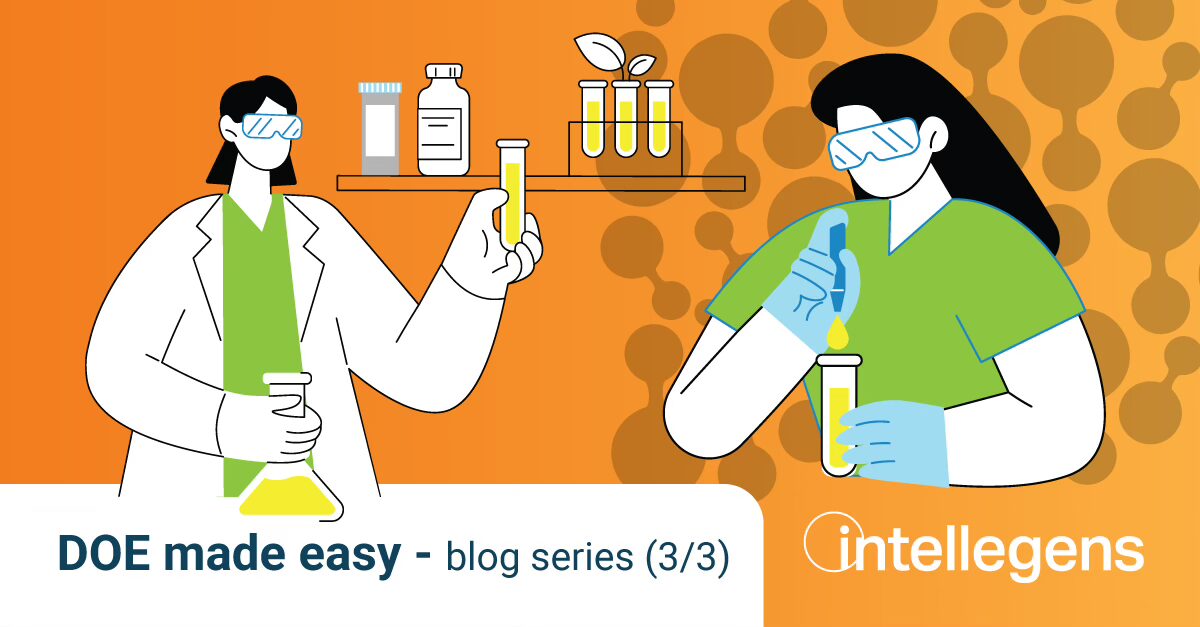
What is Adaptive Design of Experiments?
In conventional DOE, statistics are used to optimise coverage of design space while minimising the number of experiments. As we saw previously, limitations include the need to choose the best method for your scenario and that there may still be a high experimental burden.
Adaptive DOE takes a different approach. You provide target properties that you would like to achieve and an initial dataset. Machine learning (ML) learns from this data and figures out what experiments to do next in order to become more certain about how to hit your targets. You do the experiments, feed in the results, and see whether ML now confidently predicts an optimal solution. If not, you go back around the loop. This targeted, iterative method typically gets to a result with 50-80% fewer experiments.
What is needed?
Sounds great. But this approach requires special capabilities not found in generic ML tools.
Usability – any tool has to be quick and easy to use for scientists whose primary focus is setting up and analysing experiments. You shouldn’t have to learn data science principles, write scripts, or translate DOE parameters into ML-speak just to know what experiment to do next.
Dealing with real experimental data – real data is messy, often noisy or sparse (not all values in an experiment are measured). Sometimes you begin with very little data. Any ML solution must give useful answers in these circumstances, so you have a sensible starting point from which to iterate.
Uncertainty quantification – having the best possible understanding of how likely its predictions are to succeed is critical to the use of ML in adaptive DOE. This enables decisions on which experiments to do next. So your ML solution should be equipped with state-of-the-art uncertainty capabilities.
Trustworthiness – ML will always be something of a ‘black box’. It captures complex, non-linear relationships that cannot be easily described analytically. That’s a big advantage over conventional statistical methods. But scientists want to understand the basis for their decisions. So it’s important that your ML software offers explainable AI tools to dig into the relationships beneath the ML model.
Alchemite™ – DOE made easy
At Intellegens, we’d urge you to try an ML-led approach to DOE. Naturally, we’d suggest you start with our Alchemite™ solution. But, whatever tool you consider – evaluate it against the criteria set out above. Alchemite™ uses an algorithm, developed at the University of Cambridge, that works uniquely well for sparse, noisy data and employs cutting-edge uncertainty quantification. It has tools to suggest seed experiments if you have no data and a range of ‘explainable AI’ analytics.
Above all, Alchemite™ has a no-code web user interface designed for experimentalists that enables you to propose your next set of experiments in a few button clicks – DOE made easy.
The results are:
- Faster learning. ML gives recommendations based on your data, so there’s no need to learn statistical methods. No training course. No coding. Give it data, set a few simple parameters, and get started.
- Fewer experiments – adaptive DOE has the edge over conventional methods with its targeted, iterative approach. Think of it as ‘super-charged’ DOE.
- Better results – ML gives you more, because the model that it builds can be re-used predictively to conduct virtual experiments and optimise against new targets.
Interested? Why not view our recent webinar to see it in action?