Intellegens is a science-based organisation. So we’re in no doubt about the importance of the Paris Agreement. Science supports the need for the world to achieve net zero greenhouse gas emissions by 2050.
We also help organisations that make stuff – chemicals, materials, manufactured goods. And this stuff is a big part of the problem. The chemicals industry accounts for at least 7% of global emissions. Steel is responsible for a similar amount. Production of cement alone generates at least 4% of emissions.
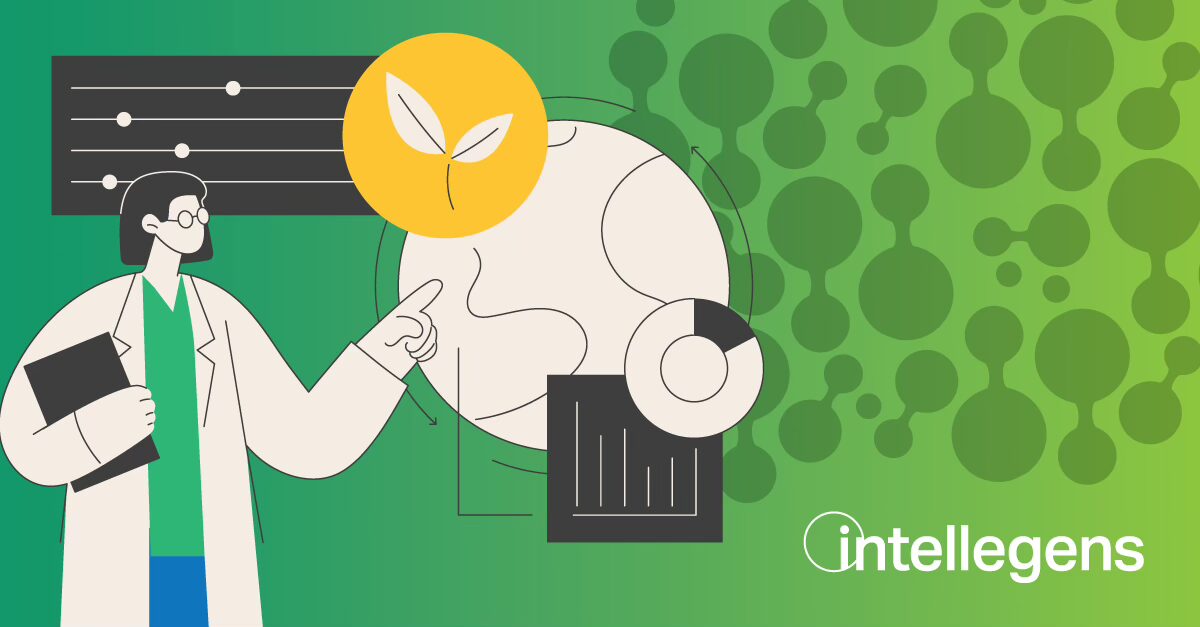
We should not pretend that this challenge can be met by just making things in a better way. Society needs to find ways to manage the rate at which we use resources, to recycle and re-use, to switch to renewable forms of energy, and so much more. But innovation is still essential, finding more efficient ways to deliver products and the built environment, while improving quality and safety so that consumers actually switch to these improved options.
There are plenty of interesting ideas around. In construction materials, for example, a recent Wired article discussed concepts including concrete that can actually capture CO2 during construction and new geopolymers that could replace existing materials. And the good news is that the companies we work with at Intellegens are highly aware of the need to make progress, fast. Almost all of the R&D teams that we talk to have explicit environmental objectives and they are challenging research partners like us to help them.
Where our machine learning technology can have the most impact is on formulation and processing. When making and using chemicals and materials, the ingredients and process steps interact in often-complex ways to determine final properties. Can we improve properties – for example, finding additives that increase the mechanical performance of a cement so that less material, and thus less energy, is required? Or can we reduce the amount of energy embedded in the material – perhaps through a lower percentage of an energy-intensive alloying element in a metal or a change in its heat treatment regime?
The clues to such changes are often hidden in existing experimental or process data. Machine learning can find relationships within this data and capture them in a model which can then be used to explore potential changes to ingredients and process parameters.
An example was the Build UK-India project in which Intellegens worked with Godrej Aerospace, Tata Steel, and Johnson Matthey to reduce waste in a manufacturing process for the aerospace industry. The project highlighted the need for excellent tools to study the subtle relationships embodied in the machine learning model. The Intellegens team added new analytics to our Alchemite™ software so that researchers can more easily see how uncertainty in an input, such as composition, translates into uncertainty in an output, such as energy consumption. This helps project teams decide where to focus their efforts as they seek to gain greater control over environmental impact.
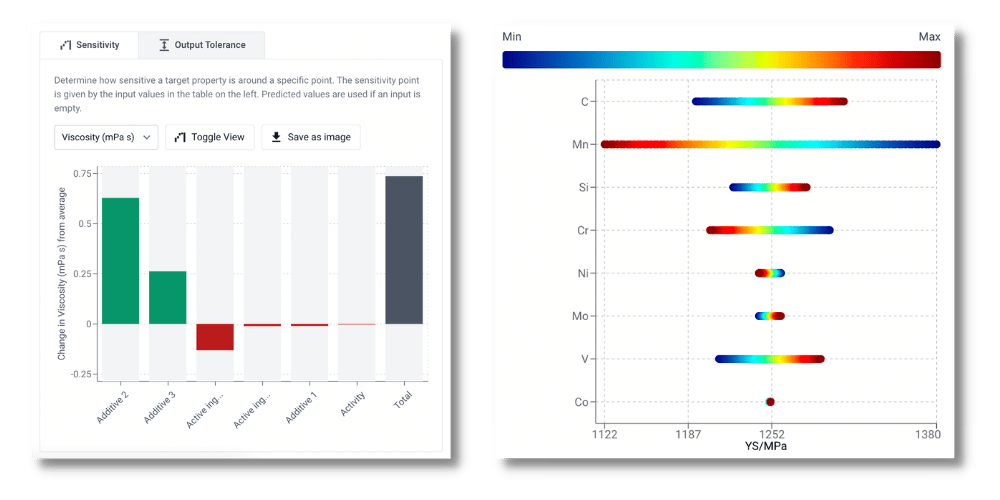
Other examples include work on concrete and on steels – in both cases, machine learning has provided valuable input into the optimisation of mechanical properties and processing. And recent work on designing new concrete formulations at the University of Cambridge included reducing the carbon footprint of the new materials. These applications will be covered in our upcoming webinar on ‘Machine learning for construction chemicals and structural materials’.
Even a tiny improvement in a large-scale industrial process can reduce greenhouse gas emissions by millions of tonnes. Tens of thousands of such processes are based on formulation problems – the type of problem where machine learning combined with the right analysis tools is a proven tool. So, while only part of the answer, we could have a big impact by formulating our way towards net zero, one process at a time.