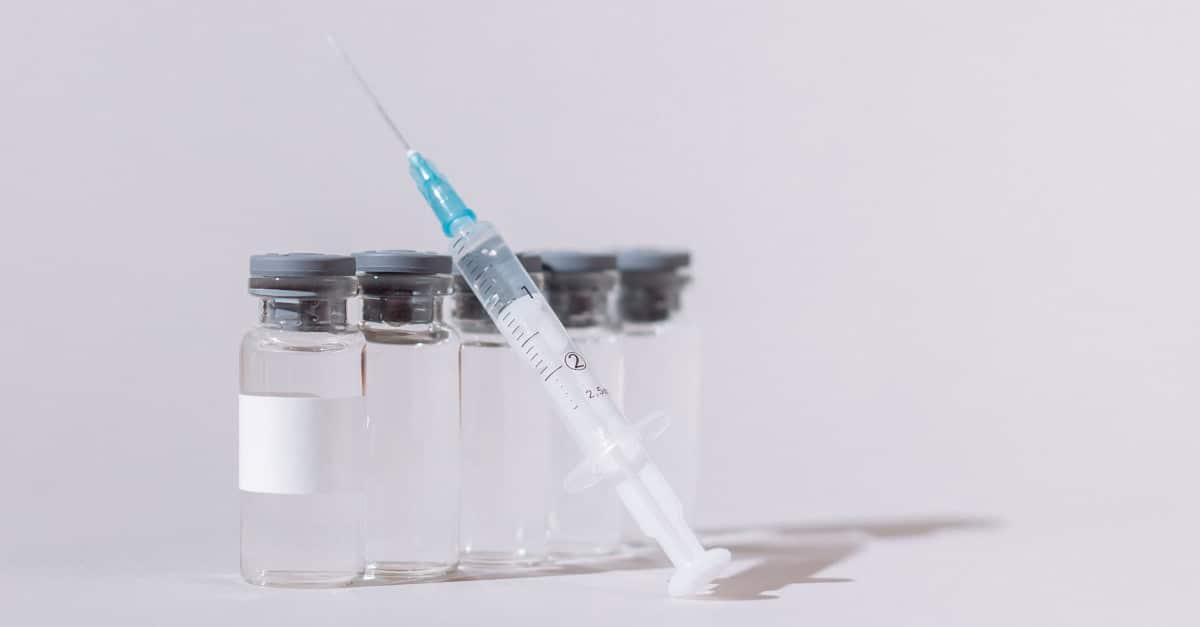
The hype machine around AI in life sciences is in full flow. New ‘AI designed’ drugs are in the pipeline. AI is discovering new antibiotics. Google is making strides in the decades-old protein folding challenge. The big consultancy businesses are writing reports with the word “revolution” in their headlines. At Intellegens, we see growing interest in applying our machine learning solutions to problems across the sector.
It’s an exciting time, and these are all positive and motivating developments. But a couple of observations seem relevant.
First, no new drug has been (or is going to be) designed and developed entirely by AI. The process still demands flashes of human intuition, smart application of chemistry and biology expertise, careful judgement, years of diligent work in the labs, translating in-silico and in-vitro successes into the human body, navigating the very human processes involved in clinical trials, and so on. What AI can do is increase the frequency of breakthrough insights, identify previously hidden relationships, and speed up many data-related tasks. In doing this, it enables some innovations that would otherwise not have been possible (which will subsequently be press-released as ‘AI designed drugs’) and, crucially, bears down on those eye-watering drug development timescales.
Secondly, AI in life science spans a spectrum from specialist, bleeding-edge R&D projects to routine data analysis. It is the former that garners headlines, and rightly so. At Intellegens, for example, we’re delighted to be involved in a newly-announced industry collaboration in the emerging area of oligonucleotides. But we will know that AI has really arrived when it is built-in to the everyday in ways that we don’t notice. Out there, in the world beyond R&D, for example, everyone is talking of Chat GPT, but no-one even notices the predictive text on their mobile phones any more.
The idea of making AI (specifically, machine learning) a pervasive R&D tool is what gets us out of bed in the morning at Intellegens. We’re deeply involved in the innovations that will be required. These include ML algorithms that can quickly and reliably generate useful insights from real (sparse, noisy) experimental and process data and tools that fit nicely into the workflows of chemists, biologists, and others in the life science R&D process. Such integration can come from embedding ML into customised tools focused on specific R&D tasks (as we have with our friends at Optibrium) but it also means making ML a data analysis tool that is as accessible and routine as a spreadsheet. Wherever there is data, there should be machine learning. This focus on data analysis has already led us to applications in drug discovery, translational medicine, clinical studies, design of experiments, and formulation (as detailed in this white paper).
“What we’ve found is that technology doesn’t supplant the people. Rather, it will enable scientists to do things faster and better,” according to Lydia The in a McKinsey report. That’s our goal for ML in the life sciences. More and better therapeutics, faster, by empowering life science R&D teams. And an industry where claiming “this drug was designed with AI” will seem as odd as it would today to claim “this drug was partly designed with Excel”.
Interested? Why not download our information pack on ML for life sciences?
White paper, datasheet, publications, videos, project information.
[salesforce form=”29″]