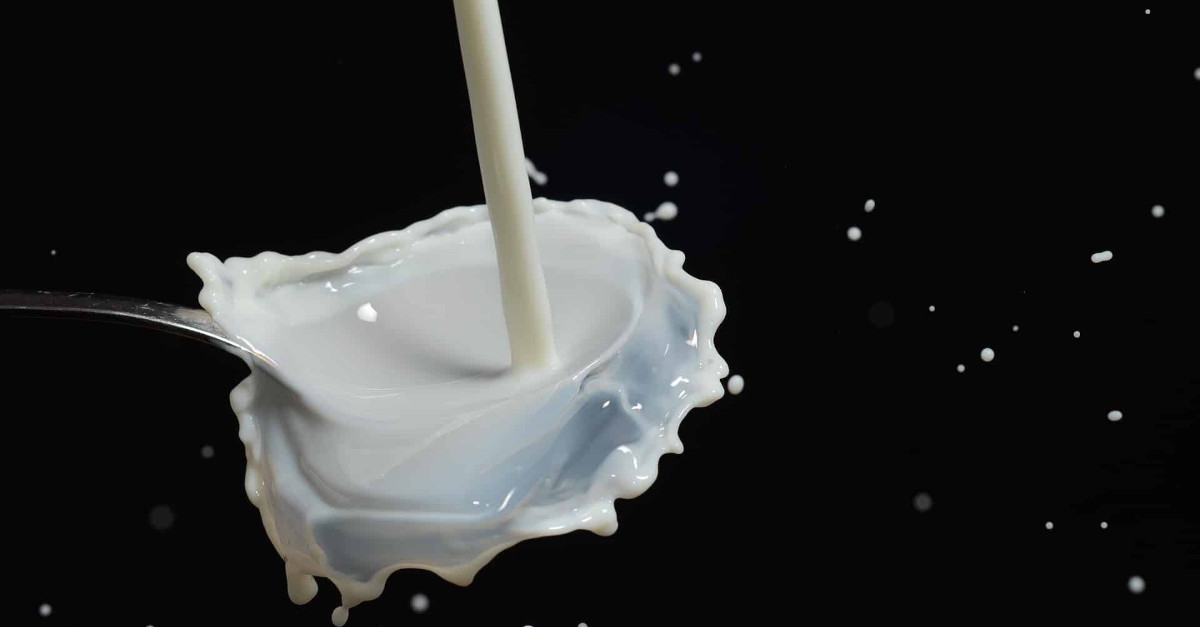
Of all the smart collaboration technologies that we use at Intellegens, the lunch table remains the most important. So, among the many fascinating applications for our software, food is one that we can really get behind as a team. The industry is top-of-mind for us for other reasons, too – we’ve seen plenty of interest in our technology from food formulators lately. So just what are food and beverage development teams using machine learning for? And, why the current interest?
The easiest way to answer the first of those questions is to point you to the webinar we hosted back in December with our friends at Yili – one of the world’s leading dairy products producers. They discussed their use of Alchemite™ machine learning to optimise UHT whipping cream formulations, mining historic test data to understand the effectiveness of various additives in extending shelf life.
When you’re in your home kitchen, a little trial-and-error with the ingredients can be fun. And the odd failed experiment is part of the experience. At least, that’s how I justified my famous lentil lasagne disaster to my family. But commercial food production is different. Large scale processes, a huge range of potential ingredients and processing options, and dozens of commercial, safety, and consumer constraints mean that the design space to be explored for food formulators can be vast, while the need for predictable outcomes is paramount. That could result in a daunting amount of time-consuming experimentation. Particularly where biological processes are in play, exact control and reproducibility of experimental conditions can be difficult. So the resulting data can be messy. Sometimes, literally.
That’s why it’s unsurprising that food producers turn to a technology like machine learning that can help them understand which ingredients and processing parameters impact the properties of their formulations, and how. And it explains why Alchemite™, with its ability to build machine learning models from sparse, noisy experimental data, might be an attractive option.
But what is driving increased interest? To some extent, this is just a product of increased awareness. Researchers are noticing more applications of artificial intelligence and machine learning in chemistry and formulation science in general, and wondering how it could help their work. But we’ve noticed a few other factors. Sustainability is a growing focus for the industry. How can they wring every drop of nutritional value from ingredients, while minimising use of resources such as energy and water? Marginal gains add up here, and so any tool that aids more rational development is valuable. There has always been a similar focus on cost, but that is perhaps heightened at a time of high food inflation. And a big driver for innovation in the sector, also driven by sustainability motives, is the emerging market for plant-based foods, including meat substitutes.
Development teams creating new or improved formulations are concerned with all of things we might expect: taste (of course!), texture, food safety ratings of ingredients, shelf life (as at Yili), cost, energy and water usage, and more. But some constraints are less obvious. One is on labelling. Regulations rightly require the ingredients of a food to be listed on its packaging. There are often practical limitations on the number of ingredients that can fit on a label. Plus, as consumers, we are naturally suspicious of very long lists. So food companies want to limit the number of different emulsifiers, colourants, and other additives in their formulations. This is the sort of real-world use case that we’ve built into Alchemite™ with the latest experimental design experience which, among other things, makes it easier to define constraints when asking Alchemite™ to propose an optimal set of formulations to test in your development process.
Despite entertaining news stories about robot chefs, AI isn’t going to take over in your kitchen any time soon. Although, in all honesty, it would not take much to improve on my lentil lasagne. But in the world of commercial food and beverage formulation, machine learning is already in use. And we should all care, because the results should be better, cheaper, and more sustainable food.
Find out more:
Case study – food formulations at Yili
Alchemite™ for formulation design