Paper published in Data-Centric Engineering
Concrete is responsible for 4-8% of all global CO2 emissions. So new concrete mixes with lower environmental impact are important research breakthroughs. A new paper in Data-Centric Engineering describes work that not only resulted in new, experimentally-validated, greener concrete, but also featured a novel application of machine learning. This was able to extract useful information from what appears to be ‘noise’ in the experimental data – taking the ability of AI to squeeze value from such data to new levels. Intellegens CSO Dr Gareth Conduit is a co-author, along with collaborators at the University of Cambridge.
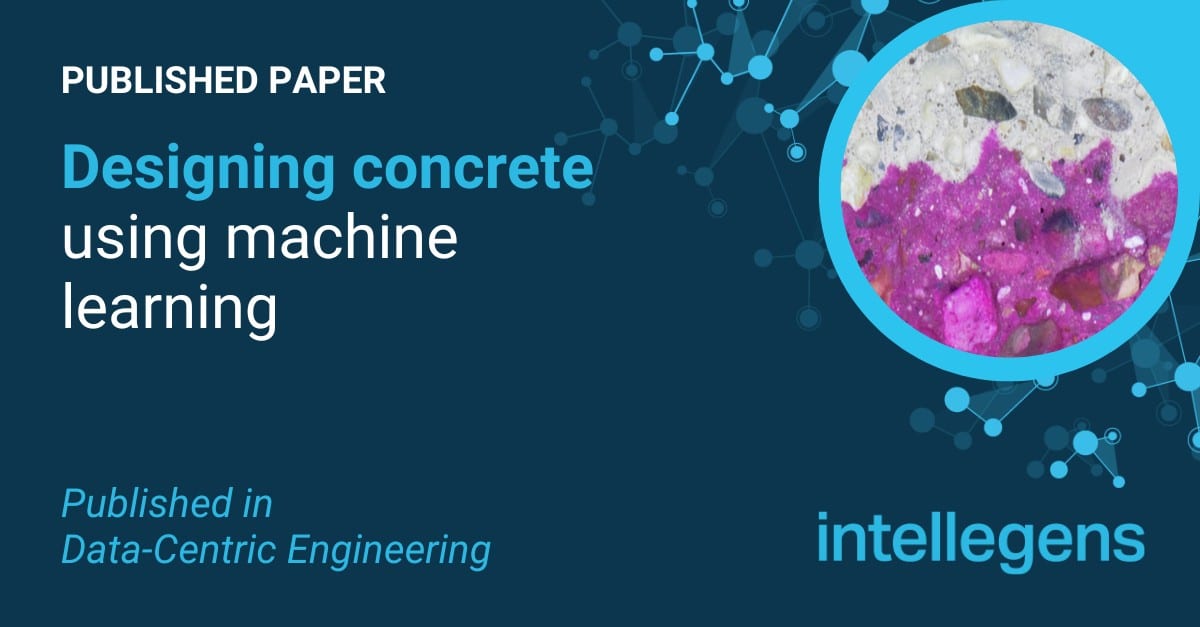
Abstract
Development of robust concrete mixes with a lower environmental impact is challenging due to natural variability in constituent materials and a multitude of possible combinations of mix proportions. Making reliable property predictions with machine learning can facilitate performance-based specification of concrete, reducing material inefficiencies and improving the sustainability of concrete construction. In this work, we develop a machine learning algorithm that can utilize intermediate target variables and their associated noise to predict the final target variable. We apply the methodology to specify a concrete mix that has high resistance to carbonation, and another concrete mix that has low environmental impact. Both mixes also fulfill targets on the strength, density, and cost. The specified mixes are experimentally validated against their predictions. Our generic methodology enables the exploitation of noise in machine learning, which has a broad range of applications in structural engineering and beyond.
Publication details
Published in: Data-Centric Engineering 4, e9 (2023)
Title: Probabilistic selection and design of concrete using machine learning
Authors: Jessica C. Forsdyke, Bahdan Zviazhynski, Janet M. Lees, Gareth J. Conduit