Paper published in J. Chem. Inf. Model.
Researchers from JTI and Intellegens have published a new machine learning study of toxicology in the Journal of Chemical Information and Modeling. Analysing experimental datasets to understand and enable control of the toxicological properties of chemicals is a key task for R&D teams developing chemicals and formulations in areas such as life sciences, fast moving consumer goods, and foods and beverages.
Such datasets are often sparse or noisy and the project compared the performance of the Alchemite™ imputation machine learning method to well-established ML-based QSAR methods, finding a significant improvement in the quality of results through the use of the imputation method.
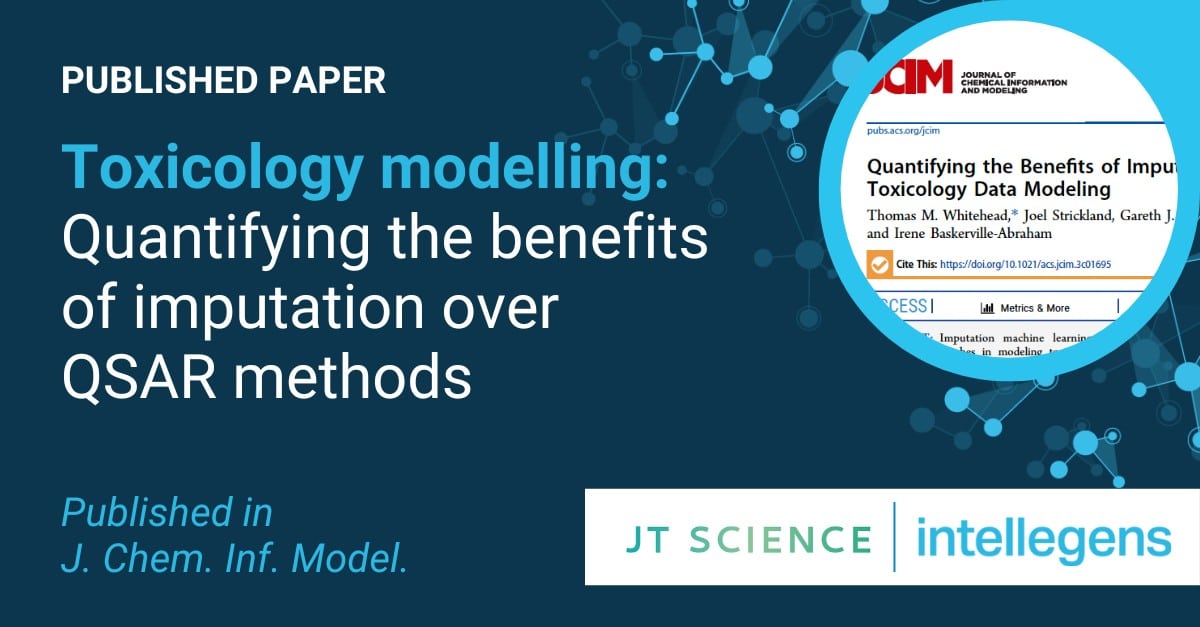
Abstract
Imputation machine learning (ML) surpasses traditional approaches in modelling toxicity data. The method was tested on an open-source data set comprising approximately 2,500 ingredients with limited in vitro and in vivo data obtained from the OECD QSAR Toolbox. By leveraging the relationships between different toxicological end points, imputation extracts more valuable information from each data point compared to well-established single end point methods, such as ML-based Quantitative Structure Activity Relationship (QSAR) approaches, providing a final improvement of up to around 0.2 in the coefficient of determination. A significant aspect of this methodology is its resilience to the inclusion of extraneous chemical or experimental data. While additional data typically introduces a considerable level of noise and can hinder performance of single end point QSAR modeling, imputation models remain unaffected. This implies a reduction in the need for laborious manual preprocessing tasks such as feature selection, thereby making data preparation for ML analysis more efficient. This successful test, conducted on open-source data, validates the efficacy of imputation approaches in toxicity data analysis. This work opens the way for applying similar methods to other types of sparse toxicological data matrices, and so we discuss the development of regulatory authority guidelines to accept imputation models, a key aspect for the wider adoption of these methods.
Publication details
Published in: J. Chem. Inf. Model. (2023)
Title: Quantifying the Benefits of Imputation over QSAR Methods in Toxicology Data Modeling
Authors: T. M. Whitehead , J. Strickland , G. J. Conduit , A. Borrel , D. Mucs , I. Baskerville-Abraham