Paper published in Computational Materials Science
A new paper from the group of Intellegens CSO Dr Gareth Conduit at the University of Cambridge applies machine learning (ML) to predict superalloy microstructure and creep properties using models that do not require their training datasets to include data on all of the elements present in the proposed alloy. This increases the possibilities for applying machine learning to study potential new alloys, providing advantages over other ML approaches and traditional predictive methods such as CALPHAD.
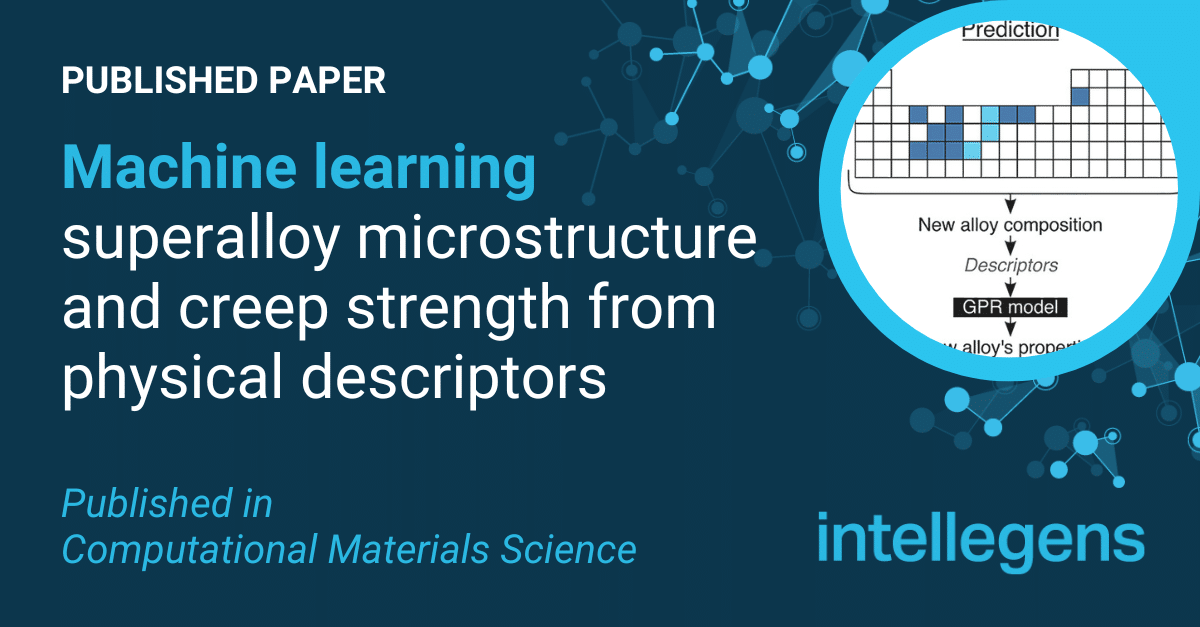
Abstract
We propose an element-agnostic set of descriptors to model superalloy properties with Gaussian process regression. Furthermore, we develop a correction method to deliver the best and most physical predictions for microchemistry in multi-phase alloys. The model’s performance in predictions is confirmed for superalloy microchemistry, microstructure, and strength properties. When including new, unseen elements in the test data, the models still give good predictions; such extrapolations into new chemical-space would be impossible with component-based descriptors.
Publication details
Published in: Computational Materials Science 227 112265 (2023)
Title: Machine learning superalloy microchemistry and creep strength from physical descriptors
Authors: Patrick L. Taylor, Gareth J. Conduit