Publication in Applied AI Letters with collaborators from Optibrium, Takeda, and Intellegens
Intellegens and drug discovery software partner Optibrium Limited, in collaboration with Takeda Pharmaceuticals, demonstrated the effectiveness of Alchemite™ machine learning for a global pharma dataset. The work is published as a peer-reviewed study in Applied AI Letters.
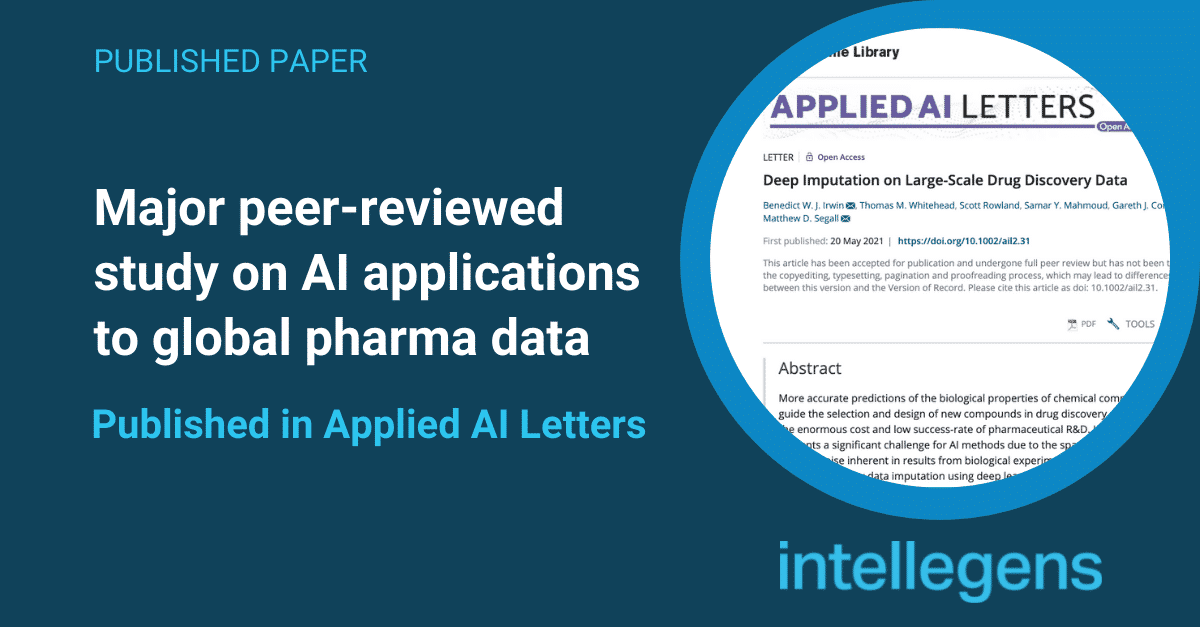
Working with Takeda Pharmaceuticals’ proprietary global dataset, the team applied Optibrium’s Augmented Chemistry® platform, demonstrating the potential of deep learning imputation to reduce cost and improve success rates of drug discovery. The platform leverages the Alchemite™ deep learning method developed by Intellegens, and was shown to deliver more accurate and reliable predictions of complex biological properties of potential drugs, enabling more effective design decisions
The study demonstrated that deep learning imputation generates new and valuable insights on global pharma-scale, high-value and proprietary datasets. Such datasets are complex, with data deriving from many different experiments, including compound activities in biochemical and phenotypic assays, high-throughput screening data and absorption, distribution, metabolism, elimination, and toxicity (ADMET) endpoints.
Furthermore, the method reliably identified the most accurate predictions on which to base decisions, which is essential to avoid missing valuable opportunities arising from inaccurate predictions. It highlighted where more experimental data are required to make a confident decision, setting it apart from other machine learning and AI methods that struggle to provide reliable confidence information on individual predictions.
Following on from a previous study, which demonstrated the effectiveness of deep learning imputation on smaller project-specific datasets, this new study showed that the same method scales to global pharma datasets. The described model was built on 1.8 million data points relating to approximately 700,000 compounds and 1,200 experimental endpoints. When applied on this scale, the insights into high-value compounds and research strategies increase exponentially.
Publication details
Publication: Applied AI Letters 2, e31 (2021)
Title: “Deep Imputation on Large-Scale Drug Discovery Data”
Authors: Benedict W.J. Irwin (1), Tom Whitehead (2), Scott Rowland (3), Samar Mahmoud (1), Gareth Conduit (2), Matthew Segall (1)
(1) Optibrium Ltd.
(2) Intellegens
(3) Takeda Oncology
DOI / Link: https://doi.org/10.1002/ail2.31