Very few scientists or engineers took their degrees because they wanted to be lawyers. They were interested in discovery, innovation, perhaps in careers where they make things work better and contribute to a greener, healthier world. And that’s where the lawyers come in, for very good reasons. Because getting to a greener, healthier world requires regulations, and scientists, engineers, and their business leaders must learn to work with the disruptive consequences.
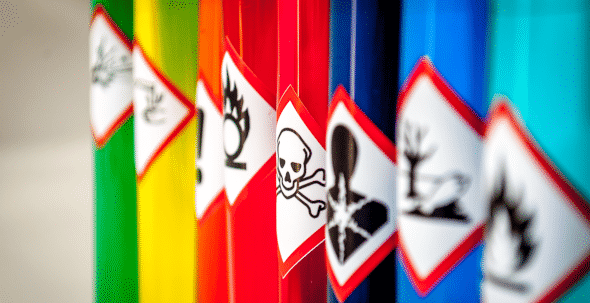
Legislation, regulations, and directives that impact the use of materials, chemicals, and related processes have proliferated in the last three decades. Back in 1986, California’s Proposition 65 set precedents by requiring companies to declare risks of exposure to harmful chemicals. Perhaps the best known example is the European Union’s REACH regulation, codified in 2006, which restricts the use of chemicals that could harm the environment or human health. The Dodd-Frank Act of 2010 tackled a different issue, placing reporting requirements on use of ‘conflict minerals’. There are hundreds of such laws globally, affecting not only companies based in the relevant regions, but also anyone selling products in those markets.
From a product development perspective, the risk is that this web of regulation disrupts a product or process that has been working well. The reasons for this, and therefore the required response, are not always straightforward. It might be that a chemical (perhaps an additive, fire retardant, or coating) is added to a list of restricted substances or materials of concern, so that it has to be removed. But the required change might be more subtle – e.g., that the substance can only be used below a certain threshold amount, or that required changes to a secondary process reduce its efficiency. Market dynamics can be changed so that a chemical that is still safe to use for your application becomes much more expensive, or that suppliers stop providing it. And changing market perceptions may mean that certain materials, even if not themselves harmful, become unpopular.
The key factors here are resilience and speed. You need to be able to change what you are doing, and fast. Yet most chemical, materials, and formulations development processes are not well-configured to work in this way. They rely on systematic studies of the candidate solutions based on experimental programs that are often slow and costly. The good news, for a business that has been working in this way for years and suddenly needs to gear-up, is that it probably has a lot of historical experimental data. And in that data, if you can extract them, may lie vital clues to where to go next.
This is where machine learning comes in. You can use existing formulation data to build models that predict how changing ingredients and process parameters impact the properties of your product. Then you can use these models to explore, for example, potential alternatives to an ingredient, how changing material costs might affect the economics of your process, or how to modify a formulation to achieve target properties while using a specified amount of an additive. The result can help you find solutions faster by suggesting ideas you might otherwise not have considered or by focusing experiments on more likely candidates, saving lots of time.
One potential issue is that legacy data used in this way is likely to be collated from different projects that were run at different times with different objectives. Such data is unlikely to form a coherent set; it will have gaps and ‘noise’. Conventional machine learning struggles to generate good results from such data. At Intellegens, we’ve developed the Alchemite™ machine learning technology to work with this real-world experimental and process data, and focused on making in work well for precisely these sort of formulation and reformulation problems. For example, Domino Printing Sciences used Alchemite™ to cut the amount of experiment required to reformulate inks.
Is your formulation, material, or chemical development process set up for resilience and a fast response to regulatory challenges? Perhaps machine learning could be a useful tool to have in your R&D toolbox?