Key outcomes
- Alchemite™ produces accurate models for kinase assay imputation
- The model accuracy is higher than traditional single target QSAR models and biological fingerprint similarity
- Accuracy plus confidence in predictions enables practical use in active learning, assay prioritisation, and potentially virtual screening
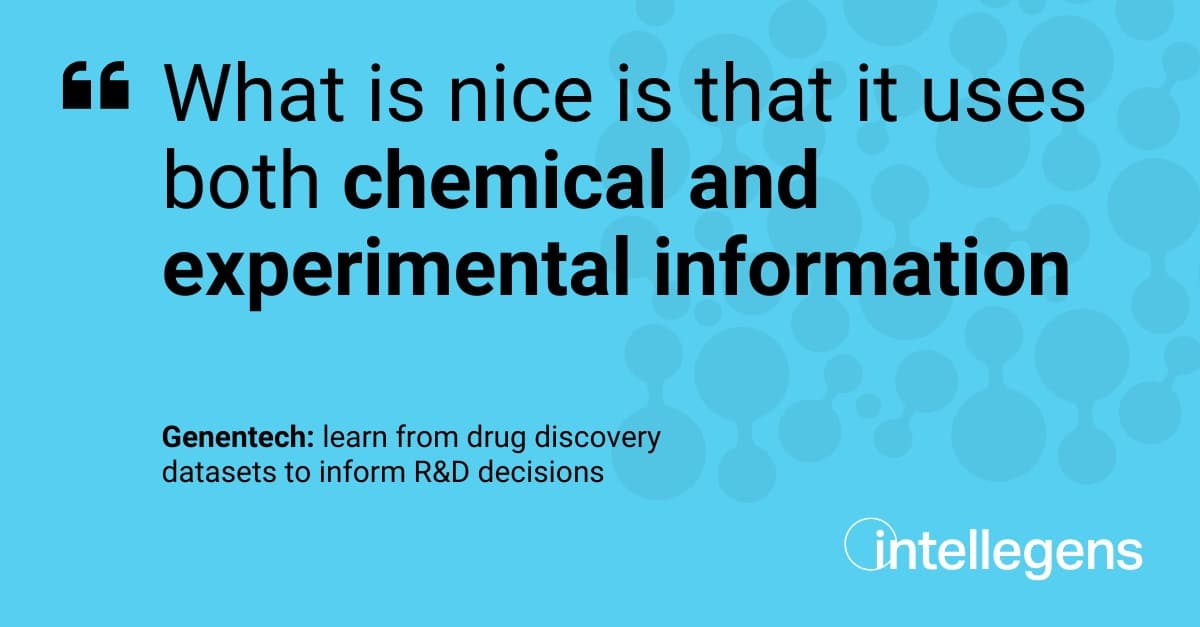
Summary
The Alchemite™ machine learning approach has been applied to optimising kinase profiling programs at Genentech. This work was described in a webinar, hosted by the Pistoia Alliance, with presentations from Fabio Broccatelli, Principal Scientist at Genentech, and Samar Mahmoud and Matt Segall from Intellegens’ drug discovery partner, Optibrium.
The team demonstrated the method’s performance on a data set of approximately 650 kinases and 10,000 compounds, significantly outperforming state-of-the-art quantitative structure-activity relationship (QSAR) approaches, including multi-target deep learning. Fabio Broccatelli commented – “Methodologically what is nice about this technology is that it uses the chemical information, but also uses the existing experimental information.”
Many computational approaches have been explored to focus kinase screening programs on key kinases while narrowing down the number of compounds and assays to run. However, they struggle with a large number of kinases and the limitation that only a relatively small number of compounds have been measured in any assay. Alchemite has unique ability to learn the interrelationships between the different kinase assays, building a model that combines the data from all considered kinases. It effectively utilises the sparse data sets available and pinpoints key kinases to predict a compound’s full kinase profile.
Related information
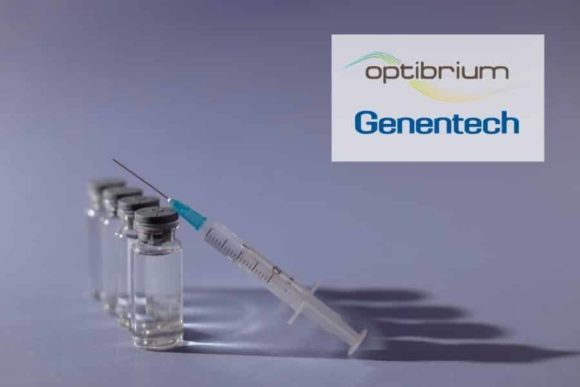