By Ben Pellegrini, Intellegens CEO
Design of experiments (DOE) seems like a ‘no brainer’. It supports innovation in chemicals, materials, and formulations while saving time and cost. In the first of this short series of blogs, we explored these benefits. In this second blog, we’ll discuss why this promise can go unrealised – and how machine learning can help.
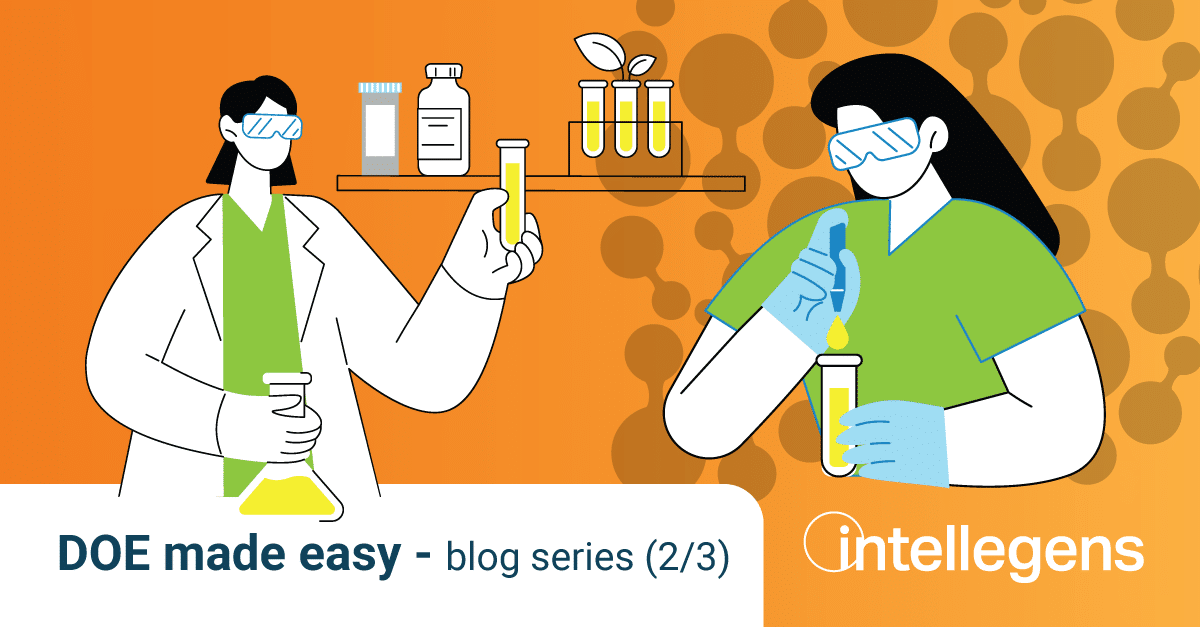
How DOE is usually implemented
Design of Experiments is rooted in statistical and combinatorial theory. By the 1980s, methods had advanced to more readily support multifactorial designs (i.e., experimental programs in which more than one factor at a time is varied) and, around this time, computer software packages for DOE began to become available – see this Wikipedia article for examples. Successors to these packages, such as JMP and Minitab, remain the cornerstone of today’s DOE practice. They have evolved impressively to provide a rich array of statistical models that apply to different experimental scenarios and extensive graphical analysis tools to probe the results – generating statistical metrics, visualising response surfaces, and so on. In today’s R&D organisations, such tools are fairly widely-used and deliver positive outcomes. Implementation usually includes a roll-out program in which potential users take training courses to understand the basics of DOE, learn some underlying statistics, and see how to apply statistical tools.
Problems with conventional DOE
Yet these DOE methods are still not universally adopted and, where they have been implemented, reception can be mixed. Here are some key challenges:
Managing change – we’ve written on this blog before about the challenge of change. R&D organisations are understandably invested in current ways of doing things, particularly where those deliver results. It is hard to shift to new approaches, even where those might mean you get better solutions, faster.
Getting started effort – DOE can be perceived as complex, and usually requires investment in that initial training. We all know the energy barrier to getting going with anything that requires a training course. With time at a premium and budgets hard to come by, organisations end up avoiding use of DOE, or restrict it to a few expert statisticians.
The need for statistical knowledge – this limitation of use to ‘expert users’ is reinforced by the fact that conventional DOE software requires familiarity with statistical methods, for example, to select which models to use for different experimental scenarios, and in interpreting the results.
Handling complex (multifactorial or non-linear) scenarios – in particular, multifactorial designs remain a complicated business, and so many DOE approaches try to limit the number of inputs varied. And DOE methods usually don’t model more complicated non-linear responses to the inputs in an experiment.
How to cover design space – fundamentally, although DOE should substantially lower the number of experiments when compared with ‘trial-and-improvement’ approaches, it is still trying to define a set of experiments that fully explore the chosen design space. Unless used carefully, DOE can still generate a relatively high experimental burden. As a user, you can limit this space, but it is hard to do this without excluding possible solutions that may not be intuitively obvious.
How machine learning can help
Machine learning (ML) can help to address these challenges:
Managing change – one key factor in supporting change is being convinced that your investment will deliver a return. ML case studies increasingly demonstrate its value, and many organisations are now testing that value through pilot projects.
Getting started effort – to support this change, we still need to lower those barriers to entry. ML can support tools that are quicker and easier to implement.
The need for statistical knowledge – one reason that ML can be made easier to use is that it ‘trains’ a model by learning from existing data. It doesn’t need the user to select a statistical approach; its model ‘self-adjusts’ to the experimental scenario presented to it.
Handling complex scenarios – ML is intrinsically multi-factorial and non-linear; it will find all of the complex inter-relationships revealed by your data and capture these in its model.
Covering design space – ML enables a process of ‘adaptive DOE’ in which, instead of trying to cover all of the available space with experiments, it understands which experiments are most likely to deliver results that approach targets specified by the user. This iterative, target-driven approach has been shown to require 50-80% fewer experiments than conventional DOE.
Using machine learning still has challenges – many of which we have addressed on this blog. What if you have very little (or no) data to start with? How do you handle uncertainty? Does ML leave room for scientists’ creativity? How can you trust the results from what seems to be a ‘black box’?
We’ll explore such questions and delve a little deeper into the ‘adaptive DOE’ approach in the final blog in this series.
Or watch our recorded webinar on DOE made easy to see machine learning for DOE demonstrated live.