Peer-reviewed Paper
This study applies the Alchemite™ algorithm to heterogeneous drug discovery data, exploring its temporal evolution to overcome the following practical issues:
- Sparse, noisy and heterogeneous data
- Training is time consuming and costly
- Overfitting and instability
- Applicability to large and small data sets
- Significant improvement over traditional QSAR models
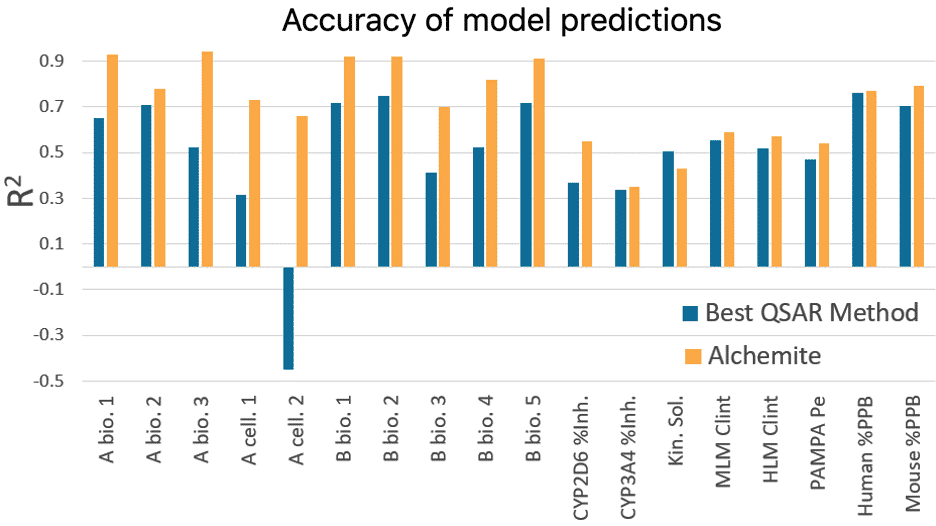
Results from this study show that, on average for an individual endpoint, Alchemite™ adds 0.22 to the R ² value of the next leading algorithm. This is possible because Alchemite™ learns from the relationships between endpoints as well as between chemical structure and endpoints, and can use this extra information to make better predictions.
Abstract
Contemporary deep learning approaches still struggle to bring a useful improvement in the field of drug discovery because of the challenges of sparse, noisy, and heterogeneous data that are typically encountered in this context. We use a state-of-the-art deep learning method, Alchemite, to impute data from drug discovery projects, including multi-target biochemical activities, phenotypic activities in cell-based assays, and a variety of absorption, distribution, metabolism, and excretion (ADME) endpoints. The resulting model gives excellent predictions for activity and ADME endpoints, offering an average increase in R ² of 0.22 versus quantitative structure−activity relationship methods. The model accuracy is robust to combining data across uncorrelated endpoints and projects with different chemical spaces, enabling a single model to be trained for all compounds and endpoints. We demonstrate improvements in accuracy on the latest chemistry and data when updating models with new data as an ongoing medicinal chemistry project progresses.
Publication details
Publication: Journal of Chemical Information and Modelling (JCIM)
Title: Practical Applications of Deep Learning to Impute Heterogeneous Drug Discovery Data
Authors: Benedict W. J. Irwin*†§, Julian Levell‖, Thomas M. Whitehead‡, Matthew D. Segall*†, Gareth J. Conduit‡§
† Optibrium Limited
‡ Intellegens
‖ Constellation Pharmaceuticals Inc.
§ University of Cambridge
DOI / Link: https://doi.org/10.1021/acs.jcim.0c00443