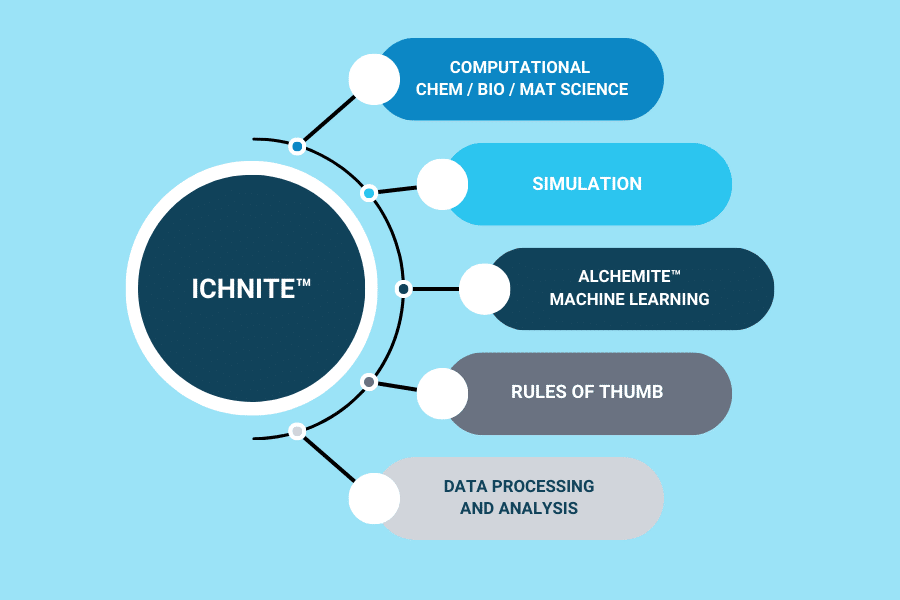
What is Ichnite™?
Ichnite™ is an application framework, executed in Python, that makes it easy to aggregate computational methods and data sources in workflows that extract deeper insights from your data. It is optimised for use with the Alchemite™ machine learning algorithm – for example, it preserves information throughout the workflow that can be used in the uncertainty quantification and analytics that are critical when using machine learning results.
Ichnite™ is typically implemented for your application in close collaboration with our Alchemite™ Success Team, delivering an integrated solution that your R&D team can apply easily and repeatably to accelerate your innovation.
Increase computational power by combining methods
Ichnite™ enables you to connect complementary computational, simulation, and analytical methods into an automated computational pipeline along with Alchemite™ machine learning. This enables ‘feature engineering’ – applying domain-specific tools to enrich data before it is analysed by Alchemite™, increasing the chances of finding valuable, relevant relationships in the data. Conversely, machine learning can be used before other methods, for example, to focus more computationally-expensive simulations on the candidates most likely to succeed.
Example applications include:
- Computational chemistry, materials science, or biology – e.g., Ichnite™ has enabled Alchemite™ to be used in combination with density functional theory calculations.
- Thermodynamic models – results from the CALPHAD method have been used to drive higher-quality machine learning predictions.
- Rules-of-thumb – integrate domain knowledge into machine learning for faster, better results.
Get value from all of your R&D data
Ichnite™ extends the range of data types that can be analysed using Alchemite™ machine learning. It can integrate tools that pre-process data into the numerical and categorical formats used as inputs to Alchemite™ and, if necessary, post-process Alchemite™ outputs to the required format. Examples include:
- Image data – for example, microstructures (case study)
- Chemical structures – for example, in SMILES format
- Time series data (read blog)
- Sequence data
- Spectra
- Text data
Federated learning: maximise collaboration while protecting IP
Federated learning is a decentralised AI approach that can be supported via Ichnite™. It brings the models to the data source on a client device for training, eliminating the costs of sharing data with the server and network latencies. This also means that a model can be trained while preserving the privacy of the data. The model can then be shared and applied elsewhere. Benefits are:
- Enables collaboration and personalisation while preserving IP: develop models from the data sources of multiple partners or clients while keeping the data secure.
- Makes efficient use of computational resources.
Customise your use of Alchemite™
Ichnite™ is a great way to get maximum value from your use of Alchemite™ machine learning by integrating it into your computational and experimental workflows in a fully-automated way. Grab results from an experimental device, process them in a computational tool that extracts key features, mix them with information from your simulation runs, then let Alchemite™ find the key relationships in the data and tell you what experiment to do next. You can even swap-out Alchemite™ for alternative ML methods to benchmark your results.
Ichnite™ + Alchemite™ = Accelerated Innovation.
How Can We Help You?
Get in touch to arrange a demonstration tailored to your application.