Where can you have too much space and yet be unable to move? It sounds like a riddle but, if you’ve ever run an R&D project, you might be familiar with the feeling.
There is a problem to solve: perhaps designing a formulation against tricky target properties. The range of potential solutions is enormous. Ingredients can be mixed and processed in tens of thousands of combinations. Analysis of the available data is not giving you much guidance. If your best option is to try everything, it feels like it’s not worth trying anything.
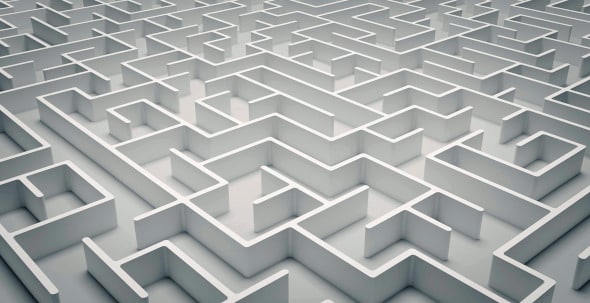
How do we create a route map for this impossible journey in multi-dimensional space? The first step (in many cases the only step available) is to extract the maximum value from the data that you have and to use that to guide you, stepwise, towards your destination. So what stops you from doing this?
A number of factors cause bottlenecks in data analysis. You might have too little data – you need to start generating more, but don’t know where to start. You might have too much data, perhaps connected to a high-dimensional problem like formulation design. A human observer, and many analysis methods, find it hard to visualize or spot patterns in large, high-dimensional datasets. Sometimes, what looks like success may miss better hidden solutions. Methods like machine learning (ML) are ideal for tackling such problems. But ML can trip up on real-world, sparse experimental data because it cannot generate useful models from training data that contains gaps.
If you can overcome that final difficulty, sparsity, then the others become easier to address. At Intellegens, we do this with Alchemite™, unique machine learning technology that can train models even when the input data is sparse and noisy. Once that hurdle is cleared, features including a fast computational engine and the ability to accurately understand the uncertainty of predictions mean that Alchemite™ can be used to impute missing data, propose optimal formulations, and suggest which experiments to do next in order to maximise knowledge for least effort. Can it break through those data analysis bottlenecks?
- Too little data – see the white paper “Alchemite™-powered machine learning with small data” for more on how this challenge is tackled.
- Too much data – working with Optibrium and Takeda Pharmaceuticals, Alchemite™ predicted complex biological properties for a global pharma dataset.
- High-dimensional problems – at Rolls-Royce, Alchemite™ designed a new aerospace alloy, simultaneously satisfying 11 physical criteria.
- Finding hidden solutions – Alchemite™ proposed an anti-malarial drug candidate that the chemists involved in the project would otherwise have dismissed.
Could machine learning help you to unpick the innovation riddle, remove those bottlenecks, and get started on your next big product breakthrough?