When we talk about machine learning (ML), we quite often focus on its ability to find patterns in data that humans will miss. That’s definitely one of its attractions. But it isn’t the whole story. Because, very often, you know things about your data and domain that will provide a short-cut to the right answers. Really, we want to marry domain expertise and AI insights to get the best of both worlds.
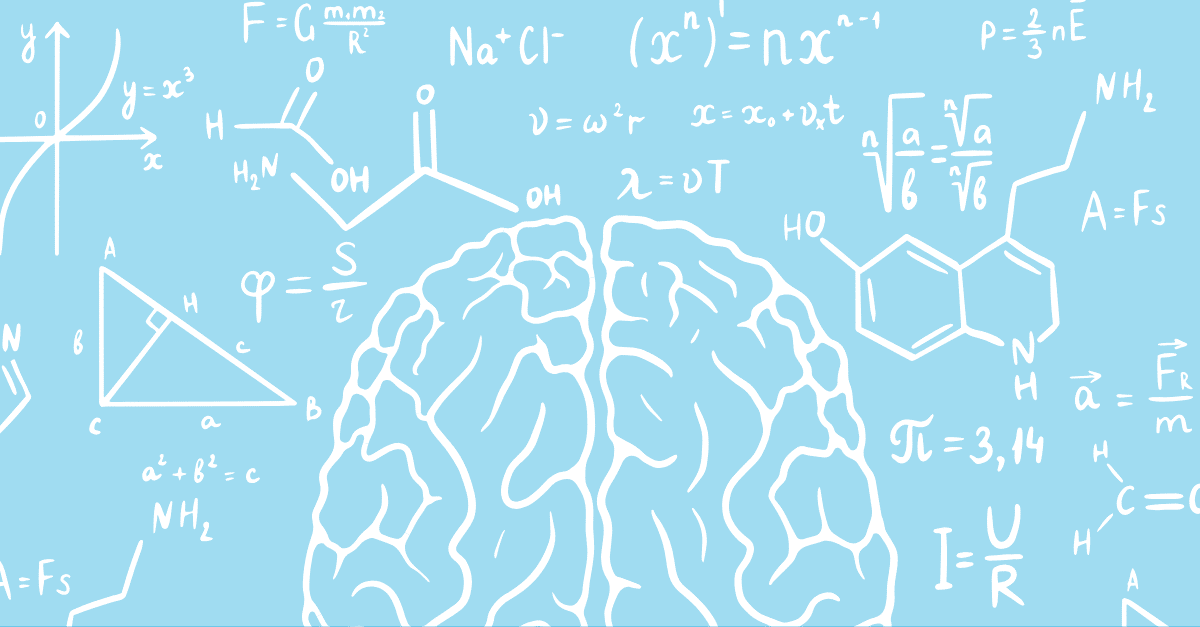
This idea came to mind when discussing some of the enhancements we’ve just made to the Alchemite™ Suite software. One of these makes it easier to add what we call ‘Calculated columns’ to your data as you use it to train a ML model. This just means that, as you upload data into the software, you can add in information about useful relationships you’re already aware of. You might know that the ratio of water to cement in a concrete formulation is best kept within a certain range, or that certain ingredients in a chemical are additives which, collectively, should be kept below a particular concentration. The new feature makes it straightforward to build in such domain knowledge before data is used to train a model. That means Alchemite™ will recommend new experiments that are more likely to work and to get you to your targets quicker.
A similar example from this latest batch of improvements is an even simpler idea. A lot of ML tools want you to clean up your data – to spend time removing comments, notes, or non-numerical data before, so that the ML only sees data that is relevant to its model building. With Alchemite™, you can now quickly identify columns containing this data as informational as you upload a spreadsheet. That information will be ignored so that it doesn’t confuse the model training process, but remains available within the Alchemite™ apps. When you come to analyze results, you can click-through and see important context for your data immediately, without having to dig out the original data – so you can make good decisions, faster, using the insight from ML alongside your experimental observations.
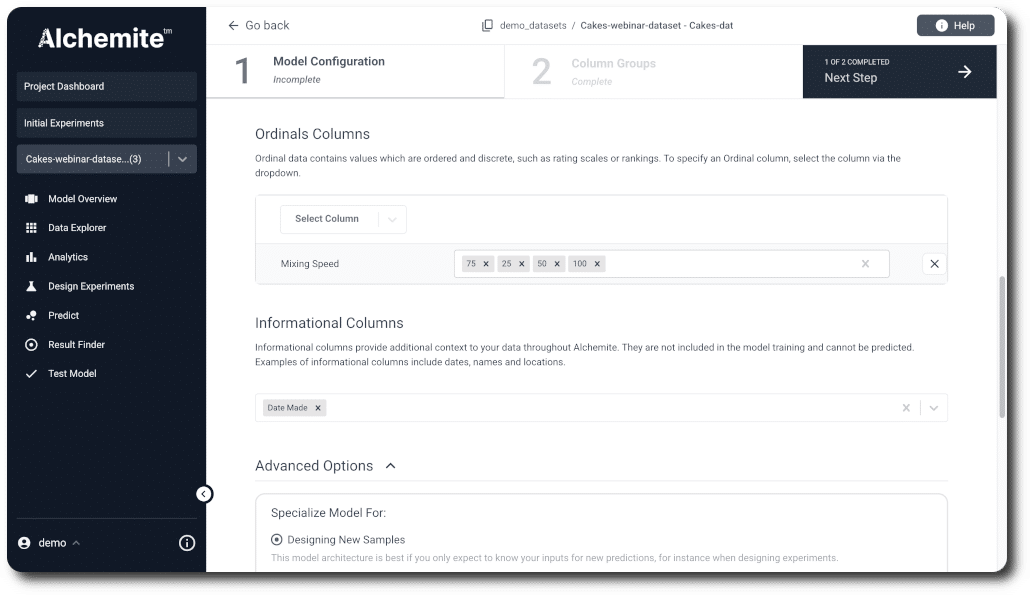
What struck me about these and some of the other features just added to the process of creating a model in Alchemite™ wasn’t their sophistication – they seem like fairly simple ideas. Rather, what’s interesting is the way that a series of enhancements like these, introduced at the right point in the workflow, really makes the technology more productive, and thus more useful. You can see this for yourself in our upcoming webinar. This isn’t about optimizing the advanced ML under the hood (although we also love doing that!) but about finding small ways to marry that AI power with scientists’ domain expertise.
Sometimes… usually… you know best. But, done well, AI can help you get more from that expertise.