“What’s under the surface?” we often ask, assuming that what really matters is not what we can see, but what is underneath. That’s usually true, of course. But what is on the surface can also be critically important. Paints, coatings, inks, and dyes beautify objects; we’ve been using them to do that for over 30,000 years. They protect against destructive uses or environments. In medical or food applications, they make objects safe to use. And they add properties, such as hardness or corrosion resistance, that could not otherwise be achieved. At Intellegens, we’re finding that machine learning can play a valuable role in the science that achieves these goals.
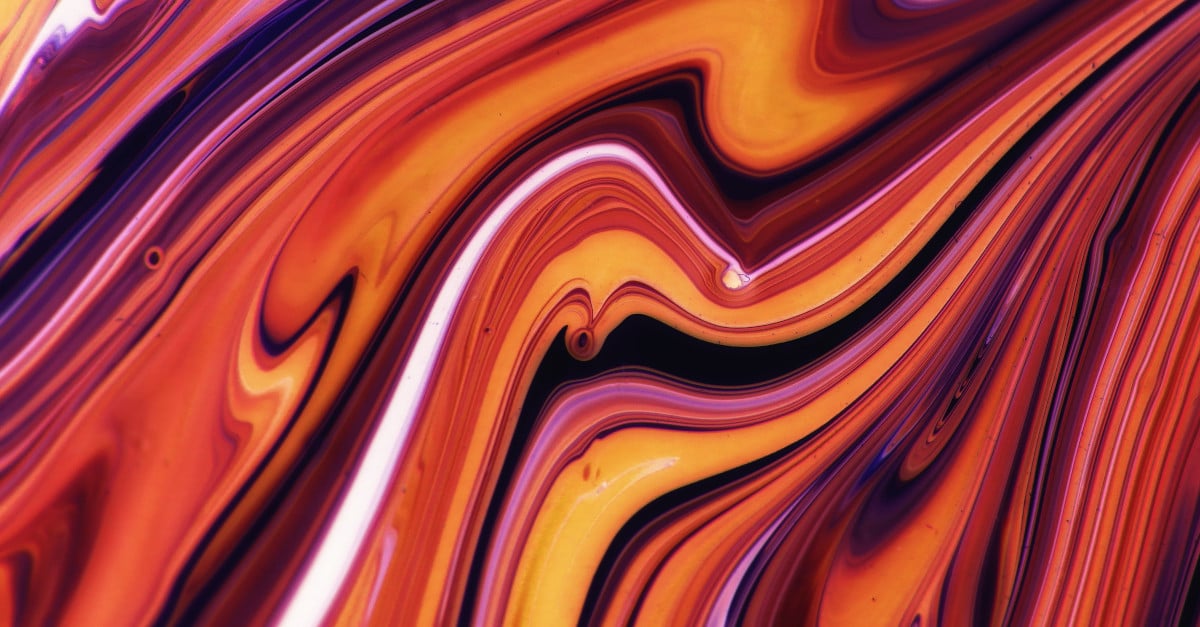
Paints and coatings are also economically important. It has always been so. In the middle ages, the ultramarine pigment derived from Lapus Lazuli was more expensive than gold, prized for providing artists with brilliant blues. Blue was also the colour when the first synthetic paint, a Prussian Blue, was discovered accidentally in 1700 – the spark for a modern paints and coatings industry that in 2021 was valued at £160bn globally.
That accidental discovery hints at something that is still true today – formulating paints and coatings is tricky. We’ve moved a long way from the literal ‘trial and error’ of early paint chemistry, of course, but the industry is still reliant on extensive and expensive programs of experimental testing.
This is because the underlying chemistry is complex, while the demands on these chemicals are increasing. A typical paint has components including pigments, binders (or resins) to hold the pigment in place, extenders to improve adhesion, solvents to control viscosity, and additives to precisely control its properties. These additives might include dispersants, silicones, thixotropic agents, driers, anti-settling agents, bactericides, and fungicides.
Of course, producers always seek to improve obvious performance metrics such as levelling (the finish on a paint), spatter, how paint sets or cures, odour, or adhesion. These properties must be optimised across a service range of temperature, humidity, and various forms of chemical attack. But the industry also needs to contend with a growing set of regulations and consumer demands relating to health and the environment – for example, to remove volatile organic compounds (VOCs) and move towards water-based solvents. And rising raw material costs and supply chain issues for speciality chemicals are increasingly significant.
This is exactly the sort of multi-parameter optimisation problem where machine learning can help. Manufacturers of paints, coatings, inks, dyes, and related additives want to mine their legacy project data for useful information and to design more effective research projects. At Intellegens, we are seeing increasing numbers of such projects. They apply adaptive design of experiment methods to explore the vast design space for paints and coatings formulations, cutting experimental time and costs. Results have included lowering production costs by finding new formulations with fewer or different additives and finding new formulations that substitute additives impacted by regulations.
If you’re working in this field, why not get in touch. If not, remember next time you paint a wall in your house that there might be a sprinkling of machine learning in the mixture!
Find out more: