Earlier this week, our Joel Strickland spoke at a webinar organised by our collaborators at CPI that highlighted the work of the UK’s Advanced Materials Battery Industrialisation Centre. This is just one example of the global push to accelerate battery innovation – a key technology in achieving net zero goals across many sectors.
Joel was discussing how machine learning-led adaptive experimental methods can be applied to speed and inform this research. From projects that Intellegens has been involved with, we can highlight three main application areas.
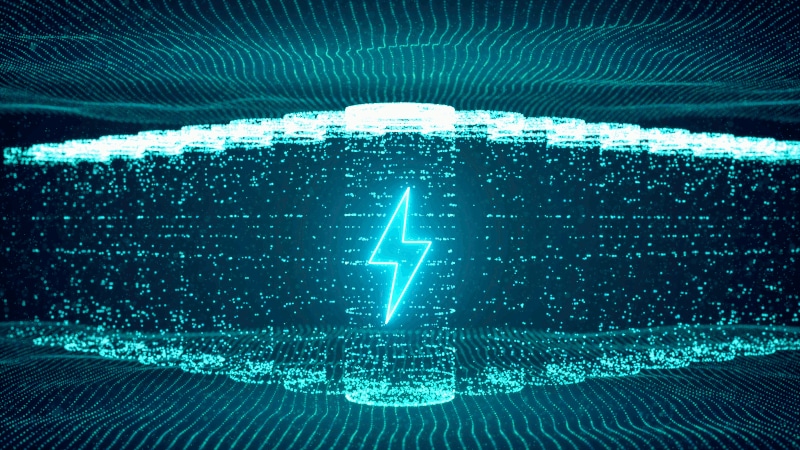
1. Intelligent materials and manufacturing R&D
This is the focus of the project mentioned above. How do we optimise the materials and formulations involved in making a battery, and the processes that create them, to improve key battery properties such as lifetime and capacity? This is the sort of multi-parameter optimisation problem, involving extensive experimental testing, where machine learning (ML) excels. ML learns from data to propose new formulation or processing options and to guide experiment to meet objectives faster, through better-targeted testing. An example is a project in which we worked with the University of Birmingham, Ansys, and the Faraday Institution to improve the design of electrodes for Lithium-ion batteries.
2. Better battery pack design
Another materials design example is discussed in our recorded webinar with battery innovator Deregellera, as is the next challenge – how you then design the battery pack itself. Here, development teams are looking for the right combination of variables such as materials, electrochemistry, cell form factor, battery pack layout, and choice of thermal management system. The aim is to control metrics such as energy/power density, cost, embodied energy, and recyclability. Again, machine learning has been applied to mine data for insights that focus research efforts, reducing costly prototyping or time-consuming multi-physics modelling in what is otherwise a huge parameter space.
3. Smarter Battery Management Systems (BMS)
Machine learning can even be applied downstream, to ensure batteries operate as efficiently as possible. Here, as the figure illustrates, ML models built during battery development using data from simulation and high throughput experiment can be used to predict key performance metrics, such as state-of-charge (SOC), state-of-health (SOH), and remaining useful life (RUL) based on the battery’s history. These models can then be applied to ‘tweak’ operating parameters on an ongoing basis once batteries are in use, so that they achieve their maximum useful life.
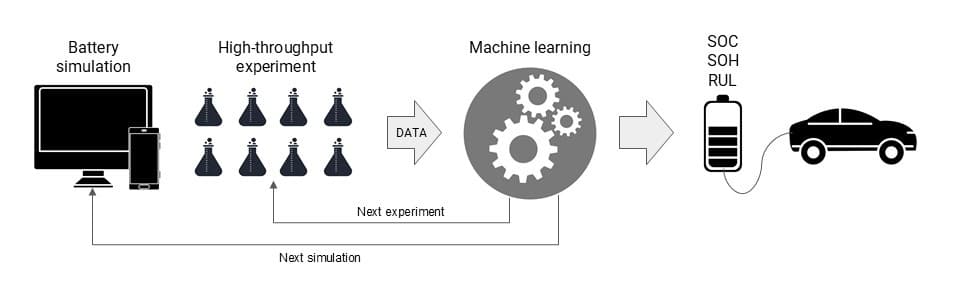
Ready to supercharge your research?
If battery research is your thing, why not read our White Paper on the topic, or get in touch, to see how machine learning could help you to more rapidly improve materials, battery design, or operation.