By Joel Strickland, Intellegens Science Team
Large Language Models (LLMs) are creating headlines, with new applications such as ChatGPT both realising the potential of AI and raising questions about its boundaries.
An increasing number of research projects have begun to demonstrate the potential of LLMs in materials science. At Intellegens, our current Machine Learning technology is based on a different type of AI – one that has already proven itself in the materials research arena. But we’re tracking this emerging technology with interest.
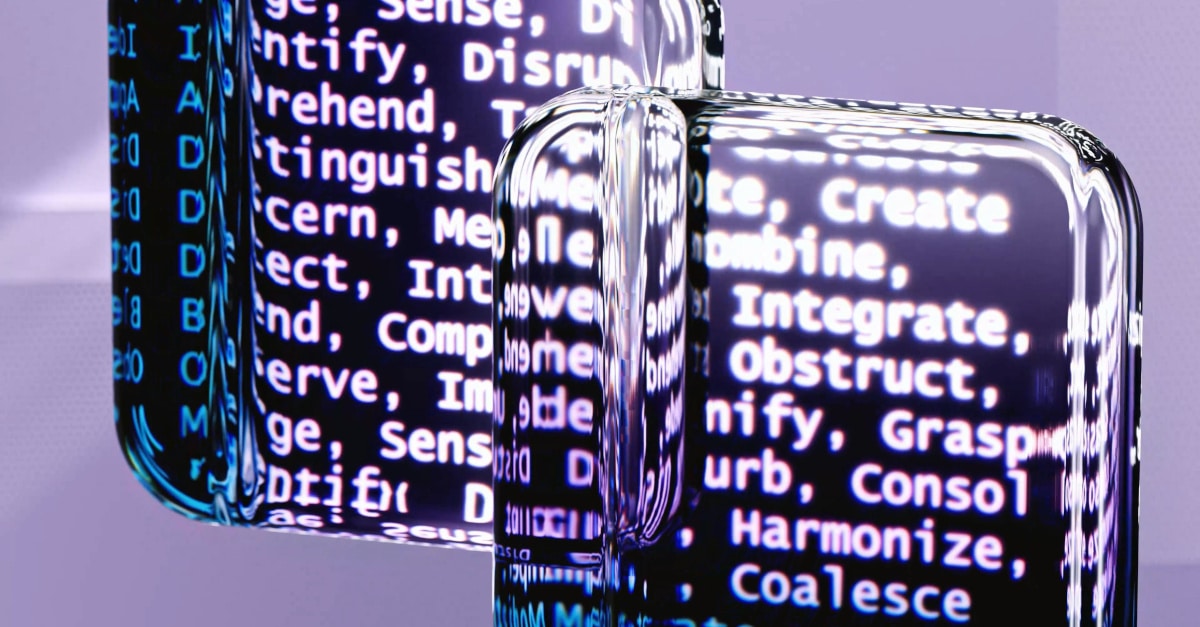
The promise of LLMs in Materials Science
LLMs, powered by their ability to process, understand, and generate language — and, more recently, images, video, and audio — are making significant strides in the field of material science. They offer a novel approach to analysing vast amounts of structured and unstructured information, uncovering knowledge hidden within those datasets, and thus enabling potential breakthroughs in material properties. Notable projects like MatChat, Coscientist, and groundbreaking research by Princeton scientists exemplify how LLMs are beginning to influence material discovery. These initiatives have successfully harnessed LLMs for tasks ranging from predicting inorganic material synthesis pathways, to improving chemical synthesis planning, to accurately predicting the behaviour of crystalline materials.
Bridging today and tomorrow
While this potential is exciting, it is still early days in the application of LLMs to materials, and significant hurdles remain. Challenges include the scarcity of specialised, proprietary data, the complexity of embedding deep material science knowledge into models, and meeting the need for the expertise required to fine-tune LLM models and develop effective retrieval/prompt frameworks.
This contrasts with other AI approaches, such as machine learning (ML), where applications to scientific data analysis are firmly established. That’s unsurprising as ML tools tend to be focused on more tractable, definable problems – such as learning from structured numerical and categorical data to predict unknown outcomes or guide experimentation. Even here, there are significant challenges, such as how to learn from complicated sparse and noisy experimental and process data – that is where the Intellegens Alchemite™ technology has been focused.
At Intellegens, we remain focused on advancing and applying Alchemite™ to materials innovation and experimental design, extracting maximum value from experimental and process datasets. But the potential of LLMs to complement this technology fascinates us too, and we are active in tracking their progress, considering how they may be useful in assisting with data mining tasks during the research projects that we support, and investigating how they might become part of practical research tools. The timescales for making LLMs a routine research tool are not yet clear, but we know that AI in general has moved with astonishing speed in the past decade, so they may be shorter than you think!
Conclusions
In the future of material science, the synergy between human expertise and artificial intelligence will open new avenues for discovery and development. Getting there will require collaboration between pioneering AI-for-R&D companies like Intellegens, developers of new methods and approaches, and academic and industrial partners at the ‘coal face’ of practical materials research. We’re already well along the machine learning leg of that journey, and we look forward to exploring the new avenues offered by LLMs.