Bogdan Nenchev from the Intellegens Science Team shares some of his work on time series.
For lab technicians, engineers, or chemists, one daunting challenge is how to design a new formulation or process in a cost effective way (with the fewest trials possible) when the collected data isn’t just isolated points, but a sequence of measurements captured over time. Classic Design of Experiments (DoE) works with data points that don’t have any sequence, but struggles with these time series. How can we tackle these problems, and why do they matter? Let’s look at a few examples.
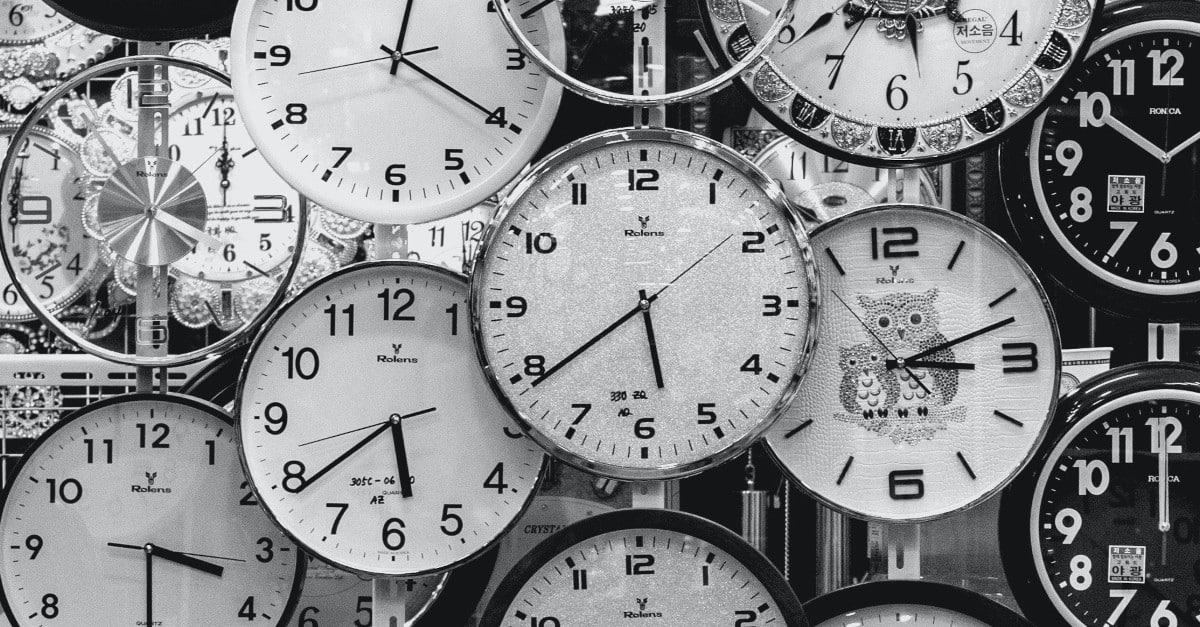
Shelf-life
For industries dealing with perishable goods, such as foods and beverages, time series data holds the key to understanding how products degrade over time. But extracting insights from this data is harder than it sounds, because models can become very complex quite rapidly once they need to account for how data measured earlier in the series may influence later results. At Intellegens, when we build a machine learning (ML) model from time series data, we are now able to establish sequential column relationships, which provide an efficient way to account for this problem. Now we can combine ingredients, process parameters, and recordings over time to predict the product shelf life. Sequential column relationships in the context of ML refers to predicting a point in time (e.g., 12 months from now) from any and only prior recorded points (e.g., 1,3, 6 months). In the Intellegens Science Team where I work, we’ve applied our Alchemite™ ML software to identify factors affecting shelf life for foodstuffs and, by using it for adaptive DoE, were able to suggest the next best formulation, additives, and processes to prolong product quality and freshness.
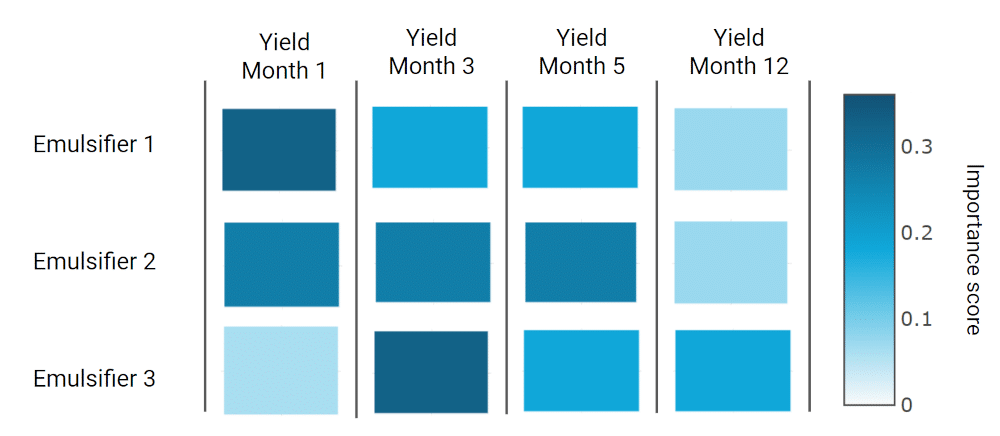
Life science: from cells to pharmacokinetics
Life-science researchers studying the growth of cells or the effects of a new drug face similar challenges. Developing drug delivery systems involves clinical trials where physiological data of human response is recorded over time. A tool we have used to study such problems is “parameterisation”. This represents a time series using a set of parameters or coefficients that capture its underlying patterns and characteristics. Such parameters are particularly relevant to this case, since they directly represent biological processes and are fully interpretable and reversible – from coefficients back to biological response.
Parameterisation, in pharmacokinetics, is akin to creating a map of known biological interactions. Take the interaction between drug absorption and elimination within a human body over time. We can apply known metrics, such as Pharmacokinetic Equations, to map the entire body response over hours-long treatment in less than five parameters. Alchemite™ can then unravel the intricate relationships between those parameters, variables and demographics, allowing scientists to spot the VIPs (Very Important Parameters) that shape disease progression, treatment response, and patient outcomes.
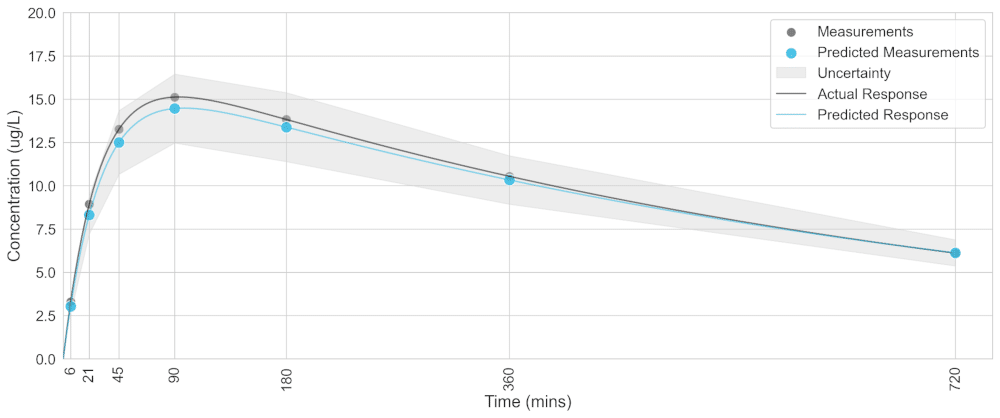
Battery technology: from wearables to vehicles
Ever wondered how the batteries in your devices get better over time? Researchers design intelligent systems that adapt to user behaviour. We have used ML to analyse user interactions with devices, gauging usage patterns, and predicting power demands.
Our Ichnite™ technology is a framework that pre- and post-processes any type of data for use in ML, including time series, images, or even molecular or genetic sequences. Our models automatically capture trends and relationships between multiple recordings over time – in the case of battery usage, these might be current, voltage, or (in a vehicle) acceleration. Ichnite™ represents the time series data in coefficients (latent space) that can be learned by Alchemite™.
Deciphering user behaviour in this way for a smartphone, a wearable device, or an electric vehicle can enable intelligent management of power consumption, enhancement of battery life, or prediction of end of life for the battery. Alternatively, applying Design of Experiments here helps researchers to configure batteries for efficiency and long life.
Time’s up
So, next time you hear about researchers analysing time series data, you’ll know they’re not just looking at complicated curves and models – they’re uncovering hidden stories that unfold over time. Whether it’s in the life sciences, material science, or battery technology this enables better decisions, creates more efficient processes, and ultimately designs better products, faster.
Find out more: