OCAS is a joint venture between ArcelorMittal and the Flemish regional government). This project:
- Extracted hidden information from microstructural images.
- Built models exhibited good predictive power, going from processing and /or microstructure to properties.
- Provided insights that enabled effective experimental and process decision-making.
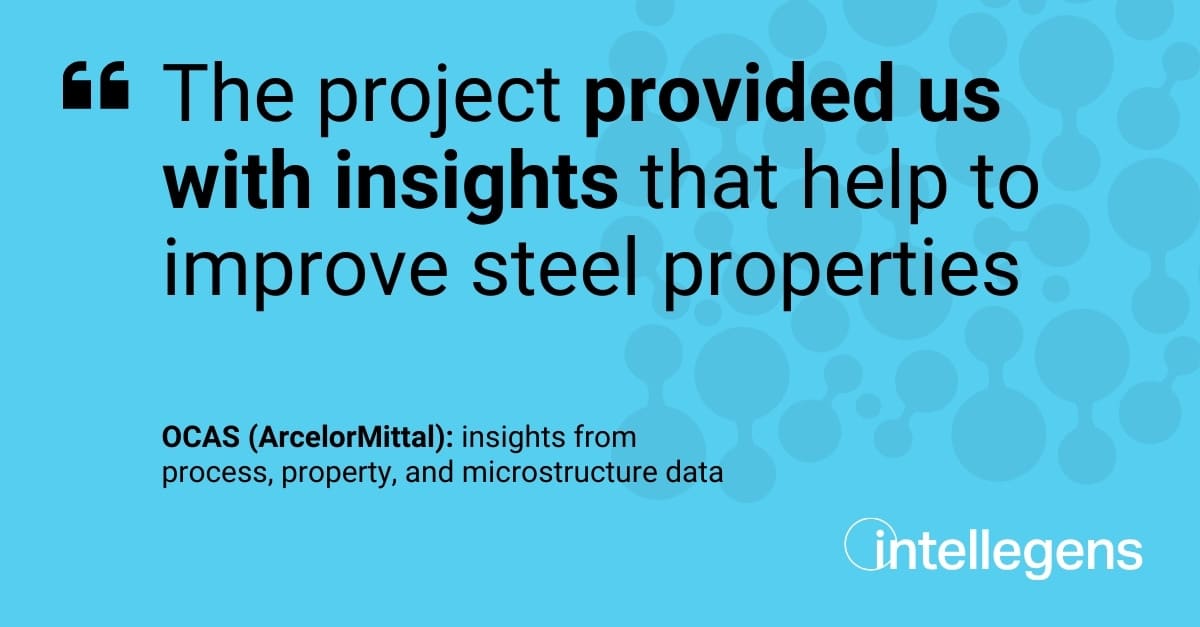
Summary
Leading steel research centre OCAS has used Alchemite™ machine learning to model the behaviour of steels. Key objectives for the project included: determining whether the method was effective for real-world, sparse data; finding out whether image data from microstructural analysis could add value to the machine learning models; and gaining insight into Processing – Structure – Property (‘PSP’) relationships.
Alchemite™ was able to build models with high accuracy, which was further improved by extracting hidden information from microstructural images. Alchemite™ models exhibited good predictive power, going from processing and /or microstructure to properties. Outputs from the project could be applied to focus valuable experimental resources more efficiently and to find new processing parameter combinations that meet specific target property requirements for steels.
Useful Alchemite™ features included graphical analytics, such as importance charts and sensitivity plots, which make the machine learning less of a ‘black box’ and reliable uncertainty estimates on predictions, which guide robust decision making. The ability to deal with sparse and noisy data was essential for extracting useful information out of real-world datasets.
Lode Duprez, chief scientific officer at OCAS, commented – “The project has validated the use of the deep learning method for real–world steels applications and provided us with insights that can help us to improve steel properties and focus valuable experimental resources more efficiently.”
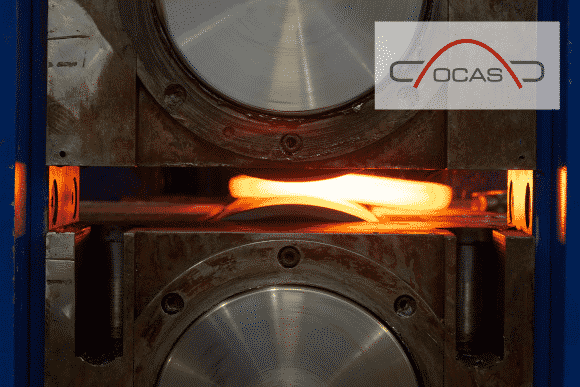