Intellegens Blog – Stephen Warde, July 2022
Discussing applied machine learning for chemicals, materials and manufacturing – see all blog posts.
Our Chief Scientific Officer, Gareth Conduit, just came back from Switzerland and what sounds like a fascinating conference on how AI can drive innovation. He brought us some nice chocolate and the observation that ‘making AI available to all’ was a recurring theme among his fellow speakers.
AI technologies such as machine learning can unlock a treasure trove of information. But who gets the key?
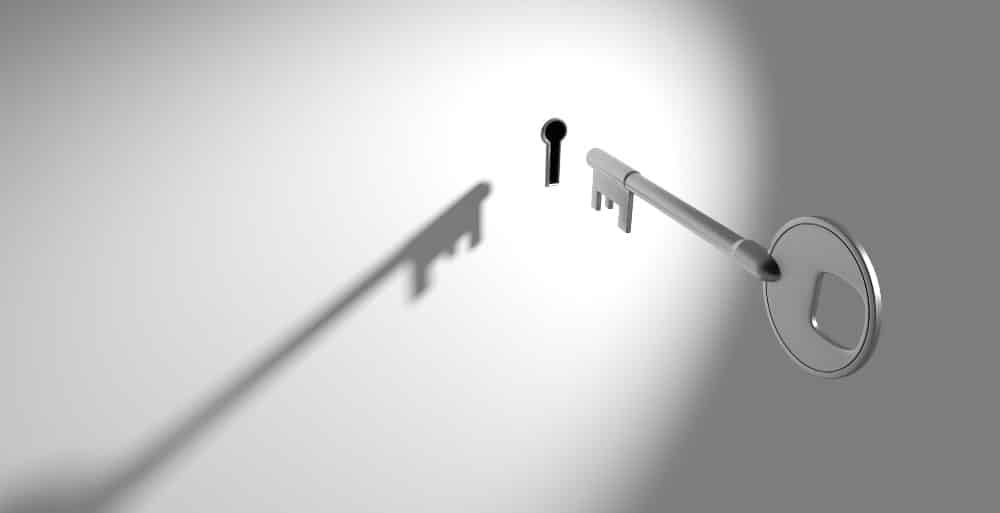
Chemicals, materials, and manufacturing organisations can see the potential of machine learning to extract value from expensively-acquired experimental and process data. But they find it tricky to apply machine learning in practice.
One approach is ‘democratisation’. Give the technology to the scientists and engineers who run experimental programs and work with this data every day. But these users can find machine learning hard to use. They need to navigate complex user interfaces or use scripting languages. And the tools often fail to build useful models from their data, which is typically sparse and noisy. Managers may also be wary of the risks of democratisation (as discussed here by Anand Rao of PwC). What if users don’t understand the technology, introducing biases into their models and making mistakes?
Another strategy is to focus data scientists on the challenge. They understand machine learning and can often manipulate data and frame problems so as to work around data sparsity. But they then find themselves spending significant effort on fairly basic data processing. They need to ask for a lot of time from their scientific colleagues to inject that vital domain knowledge into the project. And, when they deliver the results to these colleagues in the form of predictive models, the uptake is low among a community that remains wary of ‘black boxes’.
At Intellegens, we approach these issues in four main ways:
1. Handling sparsity – our Alchemite™ machine learning can build models from sparse, noisy data (as reported recently by NASA). So scientists and engineers can get started where other machine learning methods might not get to first base. Data scientists spend less time manipulating data and more time on data science.
2. The right tool for the right user – scientists and engineers apply Alchemite™ through a simple web user interface, tailored for the workflows and analysis typical of formulation, materials, chemical, and process design. Data scientists use API access to integrate the Alchemite™ algorithm with their own scripts and tools.
3. Enabling collaboration – the web platform also enables useful models to be captured and shared. So scientists and engineers can re-use experience from past projects and data scientists can distribute validated models to colleagues with ease.
4. Explainable AI – we’ve discussed previously on this blog how machine learning must not be a ‘black box’. Instead, we aim to empower scientists, providing analytics that enable them to inspect the machine learning models for insights into the physical systems being studied.
Perhaps my original question – ‘who gets the key?’ – was the wrong one. There isn’t a single ‘key’ to the treasure trove. Making best use of machine learning, as with any disruptive technology, requires not only good tools but also collaboration and a degree of cultural and process change. We need to think carefully about how to combine these elements – delivering those tools so that they improve existing skills, workflows, and knowledge. At Intellegens, we’re excited to share our experience of what it takes to run successful projects. Get in touch!
Linked to this article:
The conference ‘Discovery on Steroids: How AI will Speed up Innovation‘ was organised by the Gottlieb Duttweiler Institute, Swiss Re Institute, and IBM Research.
Rao, A. (2020) ‘Democratizing artificial intellegence is a double-edged sword‘, Strategy + Business online.
Stuckner, J. et al (2022) ‘Design of Materials with Alchemite™‘, NASA Technical Memorandum.
Product information on the Alchemite™ machine learning software.
Our previous blog on explainable AI.