We talk to a lot of R&D organisations that are considering deploying machine learning. Quite often, they’d like to get started – but not just yet. On the other hand, when we look at businesses that are a year or two into their use of machine learning, many of them wish they had started sooner.
So, let’s take a look at some of the most common reasons for delay, and why these are, in fact, often reasons to get started now.
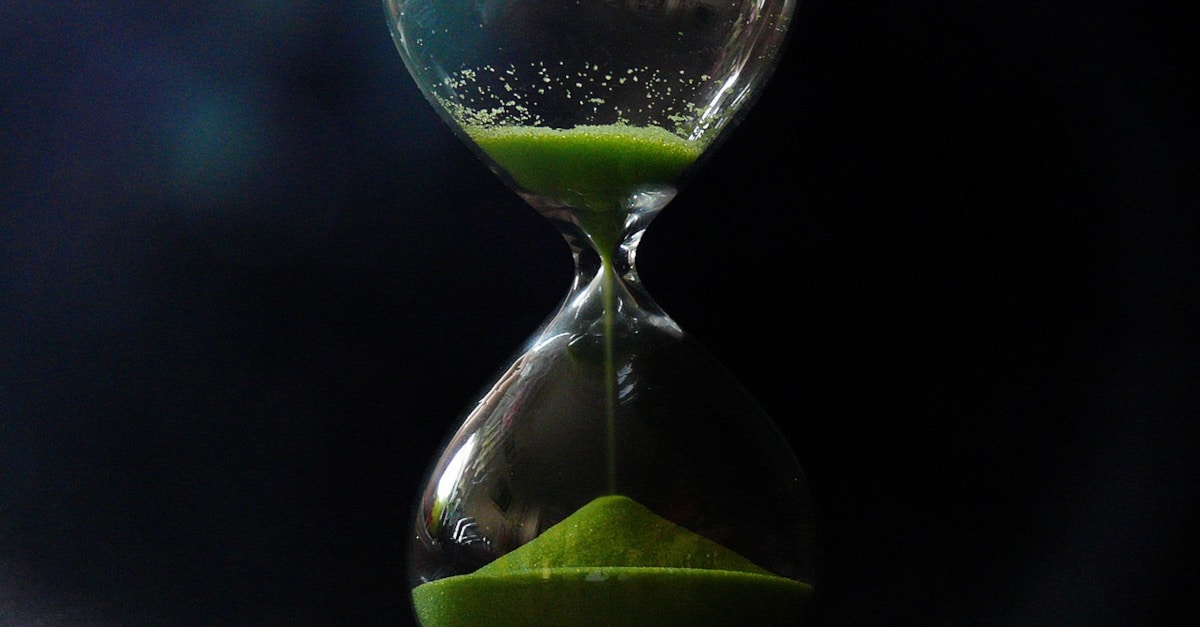
1. We need to do more experiments first
Of course, to make use of machine learning, you need data. So if you have very little (or no) data for your project, the temptation is to wait until you have generated ‘enough’. But you may be missing a trick. The earlier that you start running your data through machine learning analysis, the more value you will get from it. Models created with small amounts of data will have high uncertainties, but they can still tell you enough to focus your next round of experiments in a way that is more likely to generate data that improves the model. Use machine learning in an adaptive experimental design process from the start, and the chances are high that you will end up saving yourself a lot of unnecessary experiment.
Your reason to get started now: Experimental programs guided by machine learning are typically 50-80% faster.
2. We need to get our data organised
Getting your data organised, cleaned, and managed is a great investment. But getting the most from this process usually involves categorising and tagging data in ways that make it easier to recover and apply. Rather than waiting for the elusive ‘perfect’ database to then apply machine learning, you can use ML to help you build a better data environment. Find relationships in raw data that might otherwise be missed, giving guidance on how to classify data and on which data is most important to your R&D objectives. You may wish, for example, to prioritise some data for availability in your frontline systems and keep less important data in lower cost data archives.
Your reason to get started now: ML can help data management projects to be more efficient.
3. We need to redesign our processes to incorporate machine learning
Machine learning can be a part of developing new ways to do R&D. But it can also be used simply to make current routine data analysis tasks simpler or more efficient. And, even if you see the need for ML to be part of a wider change process, it is often best to start small and pilot its introduction within current processes, or on a smaller test case of the new process.
Your reason to get started now: Start learning as soon as possible as part of your change process.
4. We just need to see it proved for our application
Often, businesses understand the case for introducing machine learning, but want to see an example of it applied to their exact application or industry sector first. The library of case studies in different areas is growing all the time. But, if there isn’t an exact match, we’ll frequently work with scientists to understand how their detailed use case and the problems they face are closely analogous to a problem in another sector. Then there is the popular option of starting with a pilot project to validate the technology before deploying it more widely.
Your reason to get started now: You may already be behind your competition!
5. We need to build a business case
Of course, every business has processes for making investment decisions that take time and balance priorities. This is the one reason for delay that we should take at face value. But there are still some useful tips to minimise that delay. Our team can apply its project experience to help you make a business case and to create a deployment plan that minimises risk and demonstrates return on investment early. This often involves a pilot project.
Your reason to get started now: We can help you demonstrate fast return on investment.
In summary
Finding ways to get started with machine learning, often with an initial pilot project, is likely to give you earlier and better returns, and may well help you to be more efficient at those experimental, data, and planning tasks that you think you need to do before you install machine learning.