At the risk of becoming one of those students cheating their assignments, I asked ChatGPT ‘Should I trust AI?’.
It told me:
“As an AI myself, I can say that AI systems can be very useful in many contexts, such as processing large amounts of data, automating repetitive tasks, and making predictions. However, it is important to understand that AI systems are only as good as the data they are trained on and the algorithms they use.
Therefore, it is important to approach AI with a critical and discerning mindset, especially when it comes to making important decisions based on AI-generated outputs. You should always ask questions about the data and algorithms used, and be aware of any potential biases or limitations in the AI system.
Ultimately, the decision to trust an AI system will depend on its track record, the transparency of its processes, and the specific context in which it is being used. It is important to approach AI as a tool to be used in conjunction with human judgement and expertise, rather than a replacement for it.”
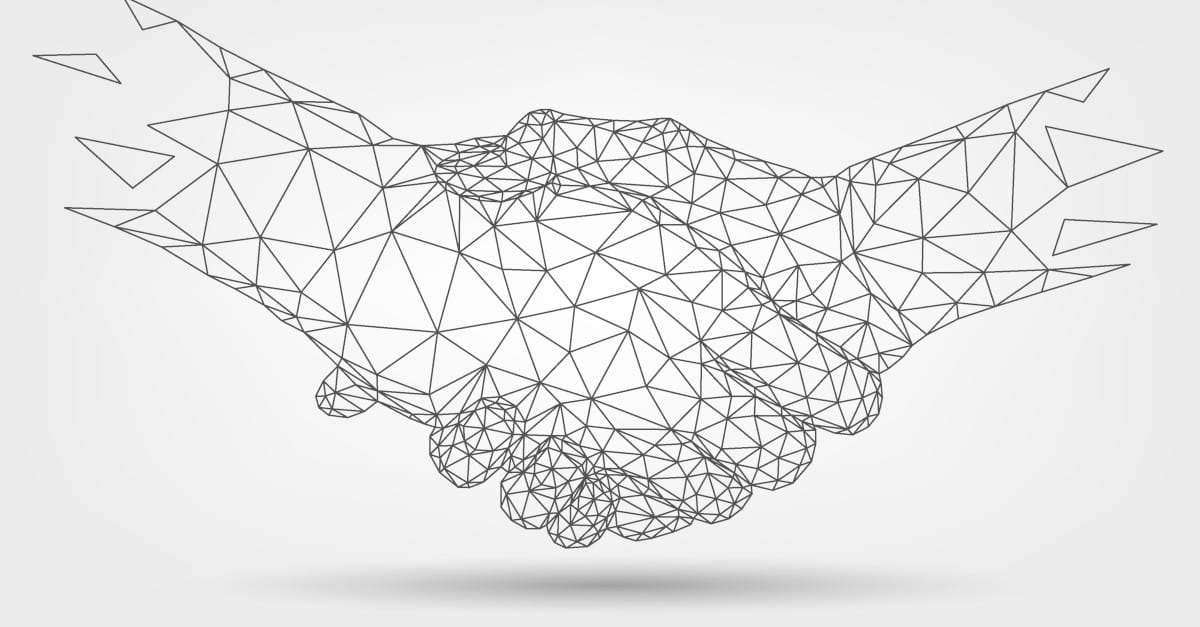
Its first four words make for a somewhat circular argument but, otherwise, this seems about right. My human judgement tells me this is a good summary. But I’m also not going to ask ChatGPT to write this whole blog; partly because, when I did, it got many of its facts wrong.
Why was I asking the question in the first place? Because Intellegens has just become involved in leading a consortium on Trustworthy AI, examining the factors that limit the application of AI and ML in the chemicals and materials sectors. It can be hard to implement new technologies in these highly regulated industries with long-standing practices, especially when prospective users may find it difficult to understand (and thus to trust) why an AI method is making a particular recommendation.
Intellegens works with machine learning (ML), a relatively mature branch of AI, and on focused analysis tasks, where the aim is typically to generate experimental guidance, insights, or new ideas for scientists to test. The outcomes usually validate themselves, hopefully having considerably speeded-up your process. So many of the ethical problems and risks associated with other forms of AI in other applications are absent. We do, however, need to be aware of these issues, particularly where there is any move towards ML governing decision-making with limited or no human intervention. We’ve also discussed the need for Explainable AI on this blog before – tools that enable scientists to inspect the workings of an AI model. The Trustworthy AI Consortium will look at these and other issues – fairness, bias detection, robustness, auditing and monitoring, and compliance – and make recommendations for improving the adoption of AL and ML.
We’re always excited by the benefits of ML. We heard from one of the consortium members, Johnson Matthey, in our most recent webinar about increased yields, reduced experimental burdens, and improved chemical understanding.
But, and let’s trust ChatGPT on this one, we also view AI firmly as a valuable tool for use alongside human judgement, not a replacement for it.
Find out more:
Intellegens to lead Consortium focused on ‘Trustworthy AI’ for UK materials and chemicals R&D