At Intellegens, we’ve just celebrated our move to a new headquarters office. So we’ve been thinking about change: its risks, how to minimise its costs, and the benefits it can unlock.
Actually, we think about these things all of the time, because we know that our customers, when they start to use our Alchemite™ software, are often committing to a change. They have a new tool to learn, perhaps a new approach to designing experiments. New understanding that needs to be developed. While scientists generally like new technologies, at least in theory, it’s fair to say that many of us also like to avoid the disruption they can bring in practice.
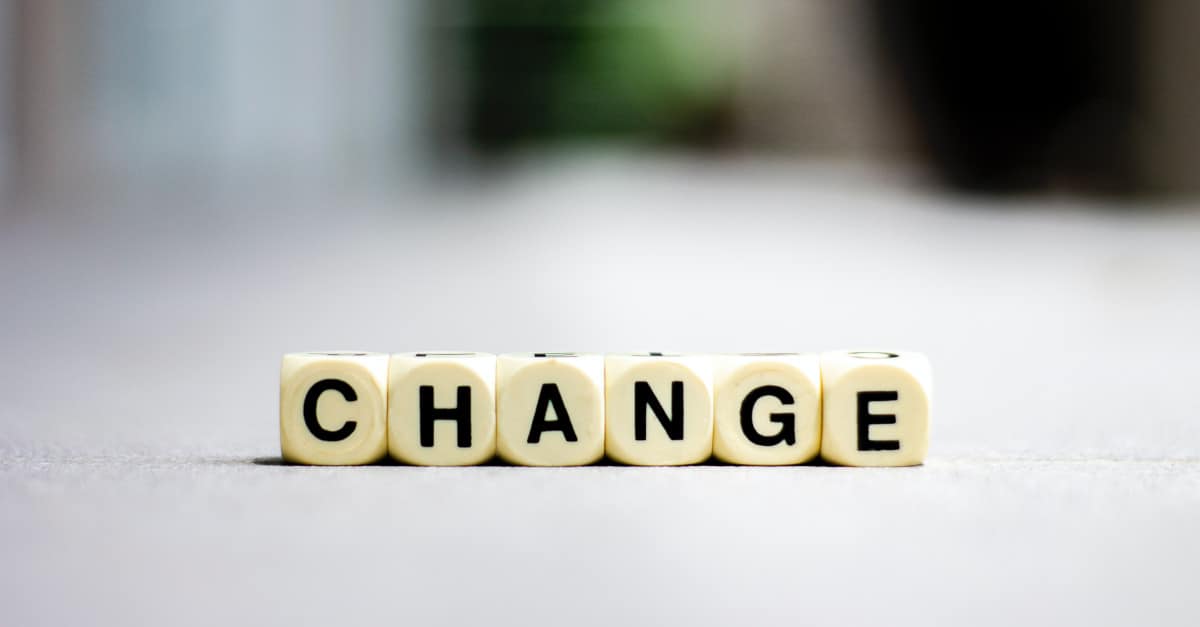
At one of our Alchemite™ Focus Group sessions we asked machine learning project leaders what, in their experience, were the biggest barriers to implementing machine learning. They cited the need, first, to think about infrastructure and data. How easy is implementation? Is the right data available? Will data be secure? How will the software integrate with existing IT systems and workflows? Next came convincing the wider community of potential users (chemists, formulators, materials researchers, engineers…) to take the time to try the tool and start using it. These scientists know some effort will be involved and, as we discussed in a recent post on this blog, can all think of reasons not to use machine learning. Our project managers told us that two factors helped here: proving the software early in the project and making it easy to use.
We’ve taken this feedback to heart, and here is how we approach each of its themes.
Infrastructure and data – We provide a web-based software system that can be deployed on the cloud or a private network. Implementation is relatively straightforward within such an environment. We pay careful attention to data security, using robust well-validated technologies, and make it simple to upload data. If limited data is available to start with, the software now has tools to recommend what experiments would most efficiently generate the information needed to get started.
Proof – We’re continually working to expand our growing library of case studies and publications. Most recently, we were pleased to host a webinar in which Gabe Guss from Lawrence Livermore National Laboratory discussed work to test the Alchemite™ software for additive manufacturing. We also take an approach to implementing Alchemite™ that typically starts with a pilot project in which we demonstrate the utility of the method for the customer’s data and application before wider roll-out. This has worked well in helping a number of companies to encourage wider adoption.
Ease-of-use – We have a strong focus on user experience (UX) to minimise the effort that a new user, unfamiliar with machine learning, has to expend to get up-to-speed. We’re pleased with the results so far. At next month’s webinar, for example, Johnson Matthey will share some of their positive experience of this usability. But we can always improve, based on feedback from customers, as with the newly-released dashboard capability which makes it much easier to evaluate the performance of machine learning models at-a-glance.
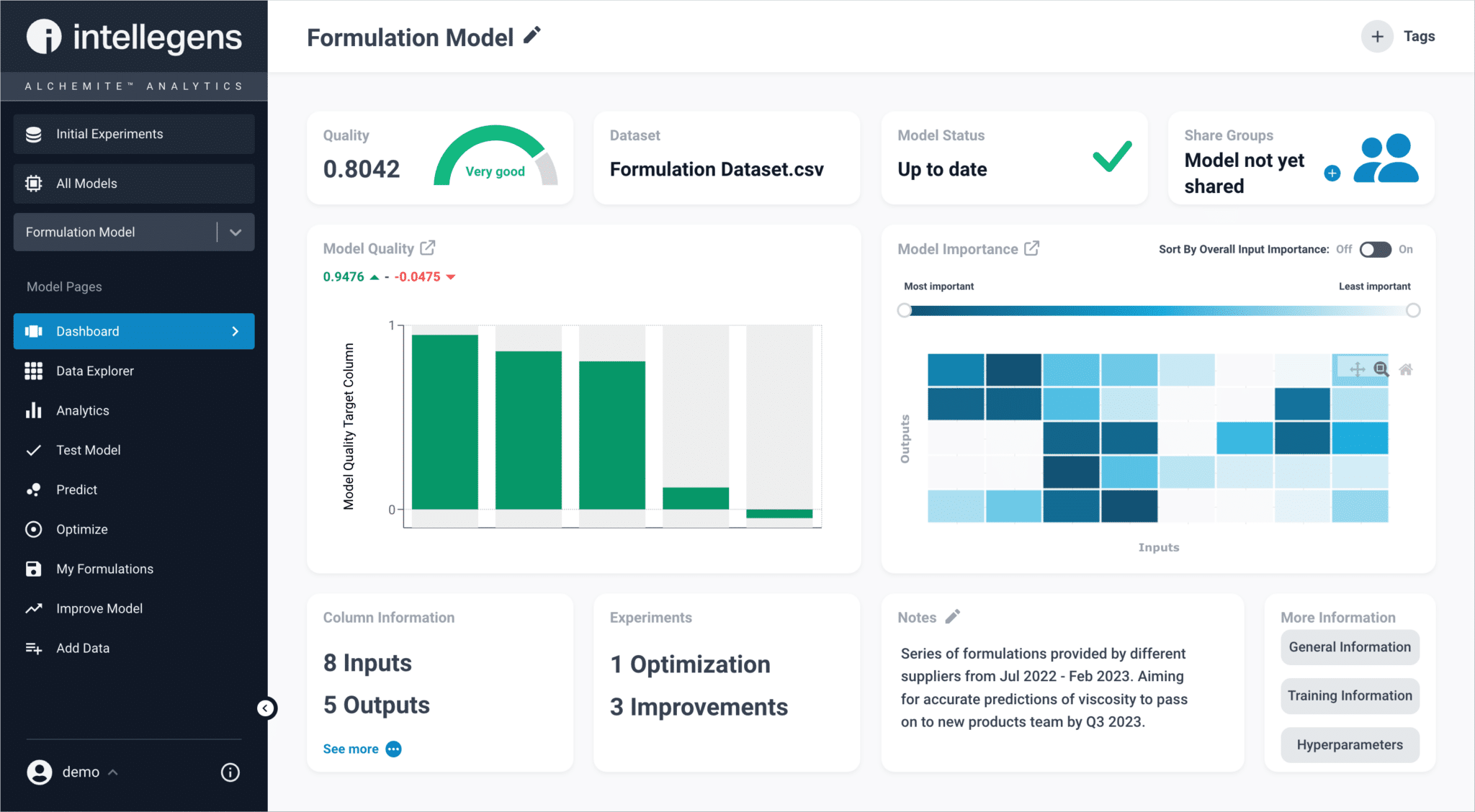
We’re very happily established in our new office now, thanks to our great admin team. They made sure the infrastructure was in place, proved that the new location was the best choice for us, and had our desks and equipment set up ready to go on day one. We apply the same spirit to software development: minimise the risk of change, maximise benefits. It takes effort. And nothing is ever perfect. We’re getting the leaky window in the office fixed. So, in software development, as in office management, we keep a sharp eye open, listen to users, and fix snags quickly.
But we’re glad we invested in change. We know it will help us to perform better in the long run. And we believe the same is true for our customers.
Key links:
Webinar – Case studies in sustainable technologies (with Johnson Matthey)