Validation Study with NASA Glenn Research Center
- Alchemite™ gives accurate predictions for use in material and component design.
- It expands data science bandwidth by generating useful insights in a single study where other methods require building of multiple models.
- Uncertainty quantification capabilities are extremely valuable in supporting informed decision-making.
- Alchemite™ can help to reduce time and cost associated with experimental measurements.
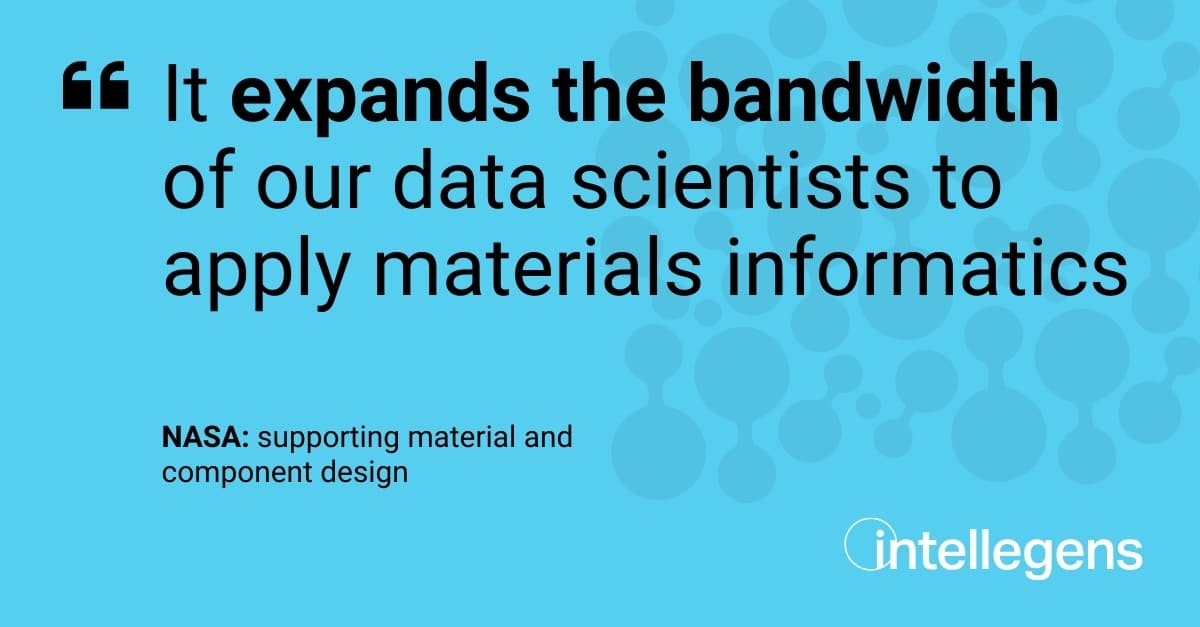
Summary
At an Intellegens webinar, Dr Josh Stuckner from NASA Glenn Research Center presented work to test and validate the Alchemite™ machine learning method. NASA had applied the tool to two projects: design of heat exchangers and shape memory alloys. In both cases, Alchemite™ was compared to alternative machine learning approaches – the presentation focused on comparisons with the Random Forest method.
Alchemite™ predictions based on complete datasets from the two applications were found to compare well with those from the other methods. But Alchemite™ offers a number of additional advantages. When data was removed to simulate typical ‘sparse’ experimental datasets, Alchemite™ performed well at ‘filling in the gaps’, while comparable methods could not function at all with sparse data. Alchemite™ can optimise over the whole of the high-dimensional space in a single study, where other methods require a different model to be built separately for each target property. This means that using Alchemite™ as a starting point for projects can save considerable bandwidth for busy data scientists, enabling them to analyse more datasets and problems. And Alchemite™ capabilities for uncertainty quantification are invaluable. These allow scientists to know how much trust to place in each predicted data point, greatly helping decision-making, in particular for safety-critical applications.
Among the practical benefits of using Alchemite™ was its ability to identify which features in the data are driving particular target properties. This enables scientists to determine which experimental measurements are really necessary in order to understand their system. This could, for example, cut the expense of microscopy analysis when microstructure features are found to be less important than other factors.
Based on the results of this validation work, NASA Glenn Research Center is integrating Alchemite™ into its inverse design architecture.
Further information
A recording of the webinar in which this work was first presented is available.
This work was published in July 2022 as a NASA Technical Memorandum, available from the NASA Technical Report Server.
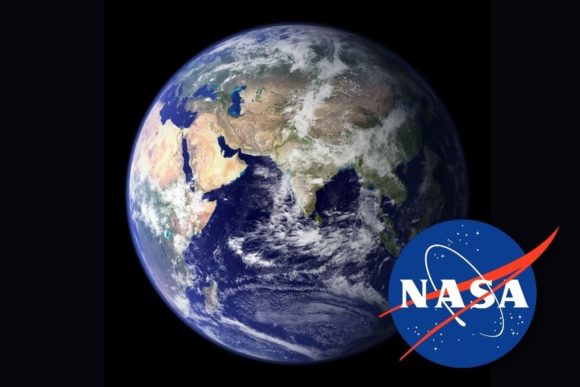