NASA applies Alchemite™ to shape memory alloys, heat exchangers
A new paper from the NASA Glenn Research Center applies Alchemite™ machine learning to the design of shape memory alloys and heat exchangers. Machine learning can enable inverse design, in which scientists start from the desired properties to determine optimal process parameters, achieving superior results with much less experimental time than alternative approaches.
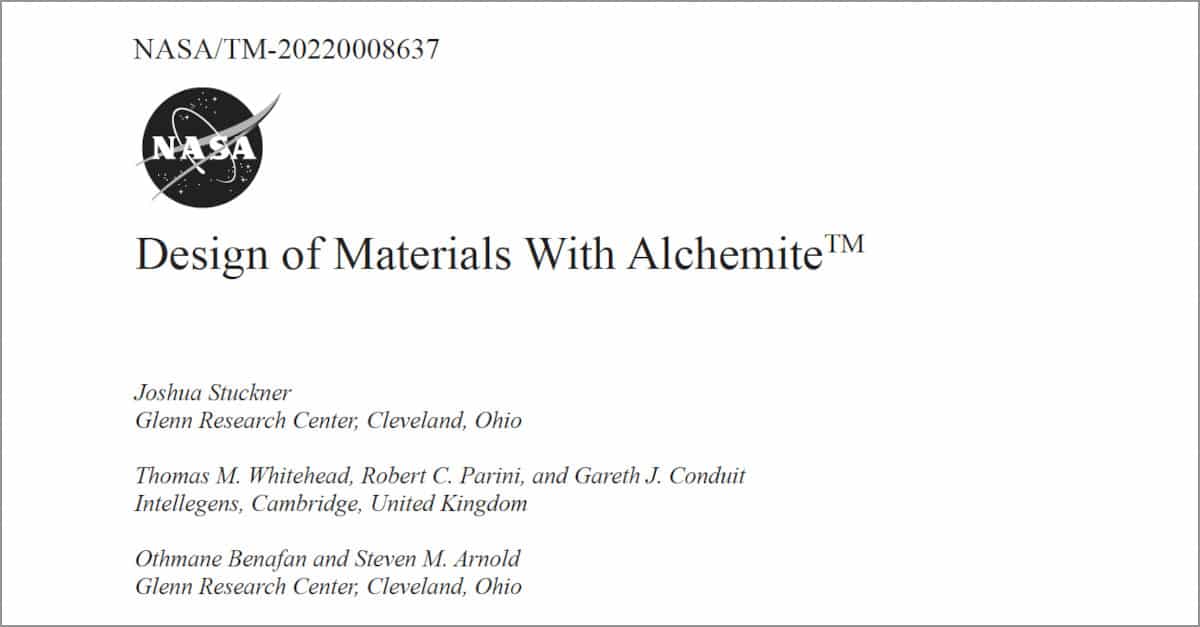
Abstract
Machine learning models that establish the relationships between materials processing and properties can enable inverse design of materials through active learning. Alchemite™ is a commercial software that can perform inverse materials design on sparse data. Here we evaluate Alchemite™’s performance on a dataset of shape memory alloys and a dataset of heat exchangers compared to baseline random forest models.
Alchemite™ had higher accuracy when making predictions on sparse data and was more accurate or nearly as accurate as random forests on complete datasets while also quantifying uncertainty. The software was also used to suggest processing steps and design parameters to optimize properties and performance; however, physical validation of the suggested design parameters was beyond the scope of this work. Several useful design insights were gained about the impact of the design parameters on properties and performance including the importance of dopant choice and amount for shape memory alloys and the importance of height and weight on the thermal resistance of heat exchangers.
Publication and webinar details
Publication: NASA Technical Memorandum 20220008637
Title: Design of Materials with Alchemite™
Authors: Joshua Stuckner, Thomas M. Whitehead, Gareth J. Conduit, Robert C. Parini, Othmane Benafan, Steven M. Arnold
The full paper is available from the NASA Technical Reports server.
This work was also discussed in an Intellegens webinar with guest presenter Dr Josh Stuckner of NASA Glenn Research Center.